Chunking is a commonly used pre-processing step when ingesting documents for retrieval in the context of AI applications. Chunking serves to divide documents into units of information, with semantic content suitable for embeddings-based retrieval and processing by an LLM.
The purpose of this technical report is to evaluate the impact of the choice of chunking strategy on retrieval performance, in a way representative of how chunking and retrieval is used in the context of AI applications.
While LLM context lengths have grown, and it has become possible to insert entire documents, or even text corpora into the context window, in practice doing so is often inefficient, and can distract the model. For any given query, only a small portion of the text corpus is likely to be relevant, but all tokens in the context window are processed for each inference. Ideally, for each query, the LLM would only need to process only the relevant tokens, and hence one of the primary goals of a retrieval system in AI applications is to identify and retrieve only the relevant tokens for a given query.
Commonly used benchmarks like MTEB take a traditional information retrieval (IR) approach, where retrieval performance is typically evaluated with respect to the relevance of entire documents, rather than at the level of passages or tokens, meaning they cannot take chunking into account.
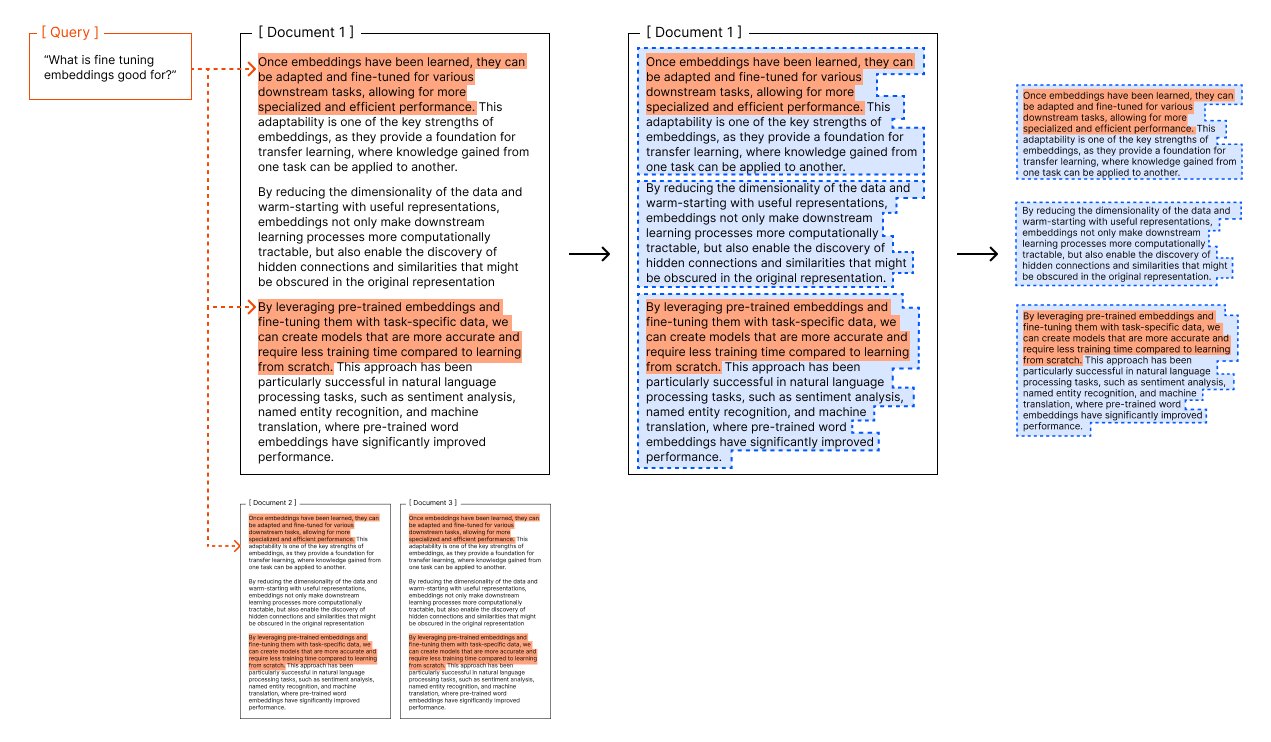
In AI applications, excerpts containing all tokens relevant to a query may be found within or across many documents. Chunks may contain both relevant and irrelevant tokens, and relevant excerpts may be split across chunks.
Traditional IR benchmarks also often focus on the relative ranking of the retrieved documents, however in practice, LLMs are relatively insensitive to the position of the relevant information within the context window. Additionally, the information relevant to a given query may be spread across multiple documents, making the evaluation of relative ranking between documents ambiguous.
Motivated by these limitations, we propose a new evaluation strategy, evaluating retrieval performance at the token level. Our evaluation uses an LLM to generate, and subsequently filter, a set of queries and associated relevant exerpts from any given text corpus, and subsequently evaluates retrieval performance via precision, recall, and intersection-over-union (Jaccard index) on the basis of retrieved tokens.
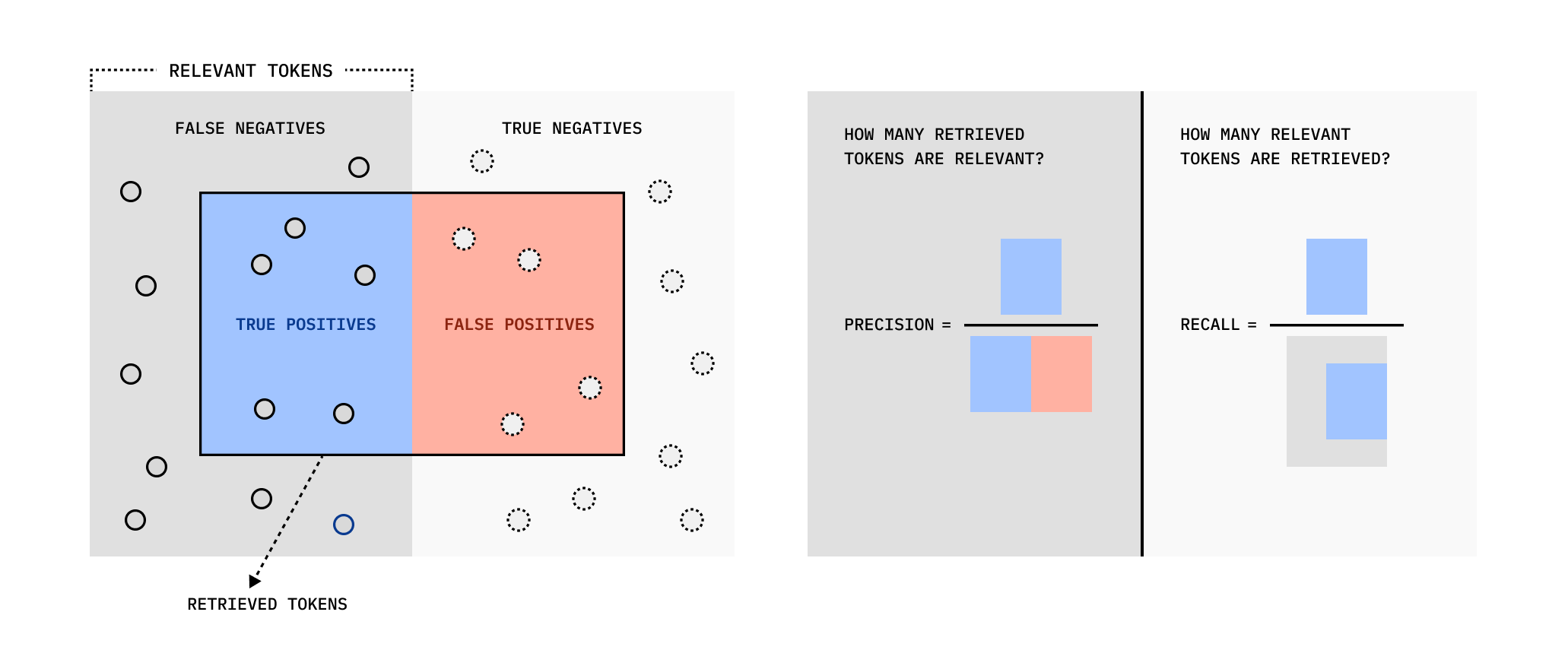
Our evaluation takes a fine-grained, token-wise approach to evaluating retrieval accuracy and efficiency, which allows it to take components such as chunking into account. The presence or absence or relevant tokens is more important than their relative ranking in AI applications.
We generate a concrete dataset across various domains with varying data cleanliness, and use this to evaluate the performance of a several popular chunking strategies. We find that the choice of chunking strategy has significant impact on retrieval accuracy and efficiency. Notably, we find that default settings for certain popular chunking strategies can lead to relatively poor performance. We also find that heuristic chunking strategies such as the popular RecursiveCharacterTextSplitter often perform well in practice when parametrized appropriately.
Finally, we propose and evaluate two novel chunking strategies; ClusterSemanticChunker, which uses embedding models directly to compose chunks up to a given size on the basis of semantic similarity, and LLMChunker, which prompts an LLM directly to perform chunking over a text corpus. We find that both produce competitive results on our evaluation.
We provide all code to replicate our results as a GitHub repo, along with useful utilities for similar experiments. We hope that this preliminary work will inspire further research into factors affecting real world performance of retrieval systems for AI applications.
Our in-depth technical report continues below. If you find our work useful, please consider citing us:
Thanks to Douwe Kiela and Chris Manning for their feedback on this work.
Interested in working on improving retrieval for AI applications? Chroma is Hiring
Introduction#
Besides answering questions and generating text [1], recently Large Language Models (LLMs) have emerged as a new type of computing primitive, capable of processing unstructured information in a "common sense" way, leading to the creation of AI applications. A key element of AI applications is so-called Retrieval-Augmented Generation (RAG)[2], wherein external data which is semantically relevant to the current task is retrieved for processing. In contrast to traditional IR, retrieval for AI applications often relies on storing and retrieving document parts (chunks), sometimes across several documents, in order to present the most relevant information to the LLM. Thus, in the AI application use-case, which tokens and chunks are returned is often as important as which documents.
Information Retrieval (IR) in general, and passage retrieval in particular, are long-studied problems in natural language processing, with a considerable literature [3][4][5]. However, benchmarks like the Massive Text Embedding Benchmark (MTEB) [6] and MSMARCO[10] do not account for token efficiency, or chunking, and usually evaluate on the basis of the relevance of entire retrieved documents. Additionally, the normalized Discounted Cumulative Gain (nDCG@K) metric, commonly used in IR [7], is less useful in the context of RAG because the rank order of retrieved documents is less important. It has been shown that as long as the relevant tokens are present, and the overall context is of reasonable length, LLMs can accurately process the information regardless of token position [8].
To address these shortcomings, we propose a new evaluation designed to capture the essential details of retrieval performance in the AI application context, consisting of a generative dataset, and a new performance measure. We describe the pipeline that generates the dataset, allowing others to generate domain specific evaluations for their own data and use cases. Because the dataset is generated, in general it should not exist in the training set of any general-purpose embedding model. Along with the dataset, we introduce a new measure of performance, based on the Jaccard similarity coefficient [11] at the token level, which we refer to as Intersection over Union (IoU) for short, as well as evaluating recall and precision at the token rather than document level. By analogy to its use in computer vision, we can think of text chunks as bounding boxes, and the IoU as a measure of how well the bounding boxes of the retrieved chunks overlap with the bounding boxes of the relevant tokens.
As an application of our new evaluation strategy, we compare the performance of popular document chunking methods, such as the RecursiveCharacterTextSplitter. We also develop and evaluate two new chunking methods, the ClusterSemanticChunker which takes the embedding model used for retrieval into account, and LLMChunker, which prompts an LLM directly to perform chunking over a text corpus.
Contributions#
We present the following:
A framework for generating domain specific datasets to evaluate retrieval quality in the context of AI applications, as well as a new measure of retrieval quality which takes chunking strategies into account.
We use this framework to generate a concrete evaluation dataset for a limited set of popular domains, and subsequently we evaluate several commonly-used document chunking strategies according to the proposed measures.
We present and evaluate new chunking strategies, including an embedding-model aware chunking strategy, the ClusterSemanticChunker, which uses any given embedding model to produce a partition of chunks, as well as the LLMChunker which prompts an LLM to perform the chunking task directly.
Finally, we provide the complete codebase for this project.
Related Work#
Current popular Information Retrieval (IR) benchmarks include the Massive Text Embedding Benchmark (MTEB) [6] and Benchmarking-IR (BEIR) [7]. MTEB evaluates text embedding models across 58 datasets and 8 tasks. BEIR includes 18 datasets across 9 retrieval tasks. The MTEB text retrieval datasets contain all publicly available BEIR retrieval datasets. Their primary metric is normalized Discounted Cumulative Gain at rank 10 (nDCG@10), and they both provide Mean Average Precision at rank k (MAP@k), precision@k and recall@k additionally.
The nDCG@K metric evaluates the relevance of K retrieved documents, giving more weight to higher-ranked documents, penalizing relevant results that appear lower in the ranking. Similarly, MAP@K takes the average of the precision at each position in the top K retrieved documents. As mentioned in the introduction, the order of retrieved documents is less important in the context of RAG. Additionally, existing benchmarks evaluate retrieval system performance at the document level, while for AI applications, only a fraction of the tokens in any document may be relevant to a particular query, and relevant tokens may be found across an entire corpus. Our proposed evaluation metrics aim to take this token-level relevance into account.
Along with information retrieval in general, there has been extensive work on passage retrieval [9], including at large scales and in a machine learning context as with the MS MARCO dataset [10]. However, this work has mostly been considered as either an intermediate step to document retrieval, or in the general context of search and retrieval, rather than in the specific context of retrieval for AI applications, and without reference to token efficiency.
More recently, LLMs have been used to directly evaluate retrieval performance, for example by prompting the LLM for a binary classification of relevancy as in ARAGOG [12]. LLMs have also been used to generate synthetic data from a text corpus, as well as evaluating the final generated output of a RAG pipeline as in RAGAS [13]. In contrast, our proposed evaluation focuses only on retrieval, with a limited step to synthesize queries from document corpora, rather than complex multi-step synthesis and evaluation pipelines which may be sensitive to model particulars and prompting. We evaluate retrieval directly, without relying on an LLM. In principle, our proposed approach can be composed with others to produce a more complete evaluation.
Despite the fact that chunking is often the first step of data ingestion in RAG pipelines, the literature on evaluating chunking strategies is sparse. Greg Kamradt's work on semantic chunking was incorporated by LangChain [14], and Aurelio AI developed its own version [15]. Additionally, Unstructured explored chunking in financial contexts, focusing on metadata about chunks based on their document position rather than optimal text partitioning [16]. These efforts highlight the emerging interest evaluations of chunking for RAG, yet there remains a significant gap in comprehensive evaluation, which our works is intended to address.
Evaluating Retrieval for AI Applications#
We introduce our evaluation framework for retrieval in the context of AI applications, consisting of a generative evaluation dataset from a supplied corpus of documents, as well as a new metric based on the well-known Jaccard similarity coefficient, token-wise Intersection over Union (IoU) which takes chunking strategies into account, and corresponds to the token efficiency of the retrieval system. We use this metric alongside other measures such as recall to evaluate the effectiveness of retrieval systems in the AI application context.
This approach to partially synthetic data generation ensures that data generated this way was not available at training time for any general-purpose embedding model, preventing possible bias. Our approach also allows for domain-specific evaluation, making it suitable for evaluating retrieval quality on any dataset. Finally, our approach can be used in combination with other evaluation approaches, including the use of human labels.
Dataset Generation#
For a given text corpus consisting of a set of documents, we sample from an LLM to generate a query relevant to the documents, as well as excerpts from the corpus which are relevant to the generated query. We present the LLM with a prompt consisting of documents from the evaluation corpus, instructions to generate factual queries about the corpus, and to provide excerpts corresponding to the generated questions.
To ensure the queries are unique, we include a random sample of up to 50 previously generated queries in the prompt. We accept only excerpts which have full-text matches within the corpus as valid. We reject any query and all associated excerpts, if any excerpt is invalid.
Query: What were the main characteristics of the armor used on the ship Atlanta? Excerpts:
- "Her armor was backed by 3 inches ( 76 mm ) of oak , vertically oriented , and two layers of 7 @.@ 5 inches ( 191 mm ) of pine , alternating in direction "
- "The upper portion of Atlanta 's hull received two inches of armor "
In order to reduce the instance of compound queries, e.g. "What was the date and significance of the Gettysburg Address?", which may complicate evaluation, we prompt the LLM to avoid using the connecting form of 'and', allowing it only when used as part of a proper noun.
For details of our prompt, see the appendix.
Dataset Prefiltering#
To ensure high quality queries and excerpts we filter the outputs of the initial generation step.
Despite prompting to avoid duplicate generated queries, the LLM will occasionally still generate duplicates or near-duplicates. We de-duplicate the generated dataset by embedding each query, and computing the cosine similarity between the embeddings of all pairs of queries. Subsequently, we filter all queries and associated excerpts where the cosine similarity is above a certain threshold value.
We note that the LLM will occasionally produce excerpts which are out-of-context or irrelevant to the query. In order to mitigate this, we embed each query and its associated excerpts, and compute the cosine similarity from the query to each of them. We filter any query and all its associated excerpts, where the query's cosine similarity with any of its associated excerpts is less than a set threshold.
The choice of threshold values for both duplicate queries and excerpt relevance is dataset dependent. We provide details of the threshold values used in the details of our concrete dataset. We use OpenAI's text-embedding-3-large as the embedding model.
We observe that the latter threshold sets a minimum cosine similarity between a query and its related excerpts. Although this might introduce a sampling bias, it is important to note that each excerpt is part of one or more text chunks, with each chunk being one among many from a given corpus. Additionally, because we perform our evaluation over multiple embedding models, the impact of this bias is negligible in practice.
We note also that although we filter aggressively to ensure generated excerpts are relevant to each query, we do not search for other exceprts in the corpus which might be relevant to the query but were not generated. This is a limitation of our current approach, which future work may mitigate by using supervised or semi-supervised methods.
Metrics#
For a given query related to a specific corpus, only a subset of tokens within that corpus will be relevant. Ideally, for both efficiency and accuracy, the retrieval system should retrieve exactly and only the relevant tokens for each query across the entire corpus.
In practice, the unit of retrieval for AI applications is usually a text chunk containing the relevant excerpt. This chunk will often contain superfluous tokens which require additional compute to process, and which may contain irrelevant distractors which may reduce overall performance of the RAG application [17]. Additionally, where a chunking strategy uses overlapping chunks, the retriever may return redundant tokens, for example if tokens from a relevant excerpt are in more than one chunk due to overlap. Finally, a given retriever may not retrieve all necessary excerpts for a given query.
We therefore seek a metric which can take into account not only whether relevant excerpts are retrieved, but also how many irrelevant, redundant, or distracting tokens are also retrieved. Inspired by a similar metric in computer vision and data mining, the Jaccard similarity [11], we propose the token-wise Intersection over Union (IoU) metric for evaluating the efficiency of a retrieval system [18].
We compute IoU for a given query and chunked corpus as follows. For a query $q$, we denote the set of tokens among all relevant excerpts $t_e$. The set of all retrieved chunks consists of tokens $t_r$.
We compute IoU for a given query in the chunked corpus as:
$$\text{IoU}_q\left(\mathbf{C}\right) = \frac{|t_e \cap t_r|}{|t_e| + |t_r| - |t_e \cap t_r|}$$
Note that in the numerator, we count each $t_e$ among $t_r$ only once, while counting all retrieved tokens in $|t_r|$ in the denominator. This accounts for the case where redundant excerpt tokens appear in multiple retrieved chunks, for example where an overlapping chunking strategy is used.
Along with IoU, we propose to use precision and recall metrics in a similar way as they are traditionally used in information retrieval, but at the token rather than the document level.
We define precision as:
$$\text{Precision}_q\left(\mathbf{C}\right) = \frac{|t_e \cap t_r|}{|t_r|}$$
And recall as:
$$\text{Recall}_q\left(\mathbf{C}\right) = \frac{|t_e \cap t_r|}{|t_e|}$$
When performing our evaluations, we report the mean of IoU, Precision, and Recall, across all queries and all corpora in our dataset. Additionally, we report precision for the case that all chunks containing excerpt tokens are successfully retrieved as Precision$_\Omega$. This gives an upper bound on token efficiency given perfect recall.
We note that we could also compute the F-score ($F_1$) as:
$$F_1 = 2 \times \frac{\text{Precision} \times \text{Recall}}{\text{Precision} + \text{Recall}} = 2 \times \frac{|t_e \cap t_r|}{|t_e| + |t_r|}$$
But in practice, IoU provides similar information while preserving a more intuitive 'bounding box' analogy.
Chunking Evaluation Dataset#
We use our evaluation framework to generate a dataset for evaluating chunking strategies for retrieval for AI applications.
Corpora#
We selected five diverse corpora for our dataset, ensuring a mix of both clean and messy text sources. Clean corpora contain well-structured text, while messy corpora reflect the unstructured nature of text typically obtained from web scraping. Each corpus is briefly described below. We provide the length of each corpus in tokens, measured with Tiktoken for "cl100k_base" (the standard used by OpenAI's GPT-4). All Hugging Face corpora are subsets of the full corpora, and the lengths provided refer to the subsets we used. These subsets always start from the beginning of the text as it is on Hugging Face.
While these corpora are relatively small in comparison to most large-scale retreival benchmarks, our aim is to demonstrate the utility of our evaluation framework on representative data, provide easily reproduced results, and to provide a starting point for future work. We do not claim that the concrete dataset we present is comprehensive, but rather that it demonstrates the utility of our evaluation framework.
State of the Union Address 2024 [19]#
This is a plain transcript of the State of the Union Address in 2024. It is well-structured and clear. This corpus is 10,444 tokens long.
Wikitext [20]#
The WikiText language modeling dataset consists of over 100 million tokens from verified Good and Featured articles on Wikipedia. Our subset is the first 26,649 tokens of this text as it appears on Hugging Face.
Chatlogs [21]#
The UltraChat 200k dataset is a high quality filtered subset of UltraChat consisting of 1.4M dialogues generated by ChatGPT. Our corpus includes all surrounding JSON syntax, making it a more accurate representation of real-world raw text. Our subset is the first 7,727 tokens of this text.
Finance [22]#
The ConvFinQA dataset is designed to study numerical reasoning in conversational question answering within the finance domain. This corpus includes complex multi-turn questions and answers based on financial reports, focusing on modeling long-range numerical reasoning paths. Our subset is the first 166,177 tokens of this text.
Pubmed [23]#
The PMC Open Access Subset is a collection of biomedical and life sciences journal literature from the National Library of Medicine. Our subset is the first 117,211 tokens of this text.
Generation & Prefiltering#
To determine the duplicate threshold, we perform a binary search over threshold values by sampling from pairs of generated queries for a given threshold, manually examine the sampled pairs, and adjusting the threshold according to whether the queries in each pair are sufficiently distinct.
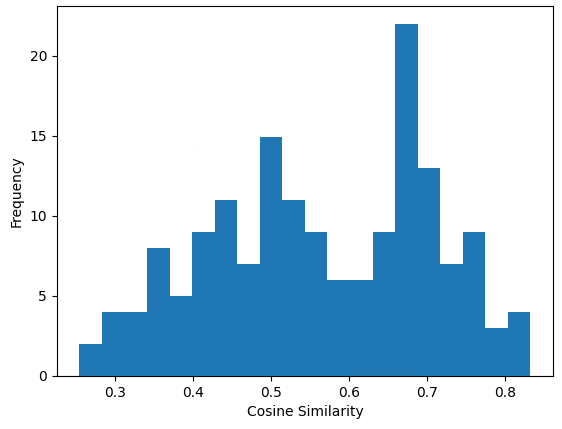
Distributions of cosine similarity between queries and their associated excerpts, Pubmed corpus.
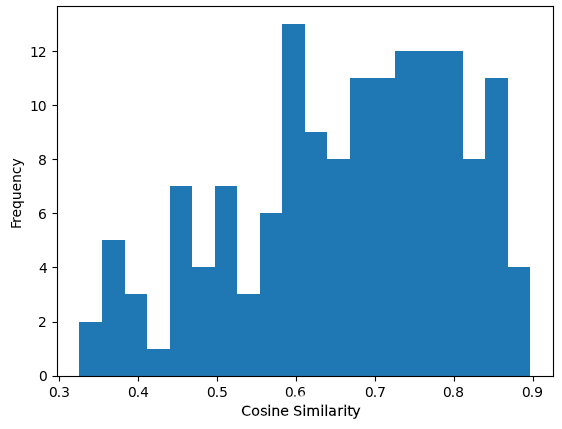
Distribution of cosine similarity between all query pairs, Pubmet corpus.
A similar approach is used for the excerpt filter. Future work could automate this process. We note also that in the adopted threshold values are similar across corpora, suggesting a heuristic value could be used in practice.
Corpus | No. of Tokens | No. of Queries | Ex. Thresh. | Dup. Thresh. |
---|---|---|---|---|
State of the Union | 10,444 | 76 | 0.40 | 0.70 |
Wikitext | 26,649 | 144 | 0.42 | 0.73 |
Chatlogs | 7,727 | 56 | 0.43 | 0.71 |
Finance | 166,177 | 97 | 0.42 | 0.70 |
Pubmed | 117,211 | 99 | 0.40 | 0.67 |
Total | 328,208 | 472 |
Number of tokens, number of queries, excerpt threshold and duplicate query threshold for each corpus.
All text excerpts and queries are available in the GitHub repository.
Time & Cost Analysis#
The time required for query generation varied between 3 to 16 seconds per question, averaging around 10 seconds. The longer times were primarily due to the need to disqualify questions based on invalid excerpts, which required additional processing to generate valid questions.
For our base prompt, we used 703 tokens (measured with OpenAI's cl100k Tokenizer). During query generation, we included a random 4,000-character subset from the original corpus and 50 sampled queries from the dataset, resulting in an average prompt size of 2,000 tokens. The average response length was 200 tokens, giving an overall input length of 2,000 tokens and an output length of 200 tokens per question.
At the time of writing, the cost of generating a single question using GPT-4 is approximately \$0.01. Consequently, creating a dataset of 1,000 questions would cost around \$10. Note that this estimate does not account for the subsequent filtering steps, which would reduce the final question count.
Chunking Algorithms#
Below we present the chunking methods used in this report. We evaluate general-purpose, commonly-used chunkers, as well as novel approaches . Any mentions of tokens in this section refer to that within the context of OpenAI's cl100k Tokenizer. The symbol ★ indicates that the subsequent algorithm is a new chunking method we developed for this study.
RecursiveCharacterTextSplitter & TokenTextSplitter [24]#
RecursiveCharacterTextSplitter
and TokenTextSplitter
are some of the most popular chunking methods, and the default used by many RAG systems. These chunking methods are insensitive to the semantic content of the corpus, relying instead on the position of character sequences to divide documents into chunks, up to a maximum specified length. We follow the implementation of the popular Langchain library.
We found that it was necessary to alter some defaults to achieve fair results. By default, the RecursiveCharacterTextSplitter
uses the following separators: ["\n\n", "\n", " ", ""]
. We found this would commonly result in very short chunks, which performed poorly in comparison to TokenTextSplitter
which produces chunks of a fixed length by default. Therefore we use ["\n\n", "\n", ".", "?", "!", " ", ""]
as the set of separators.
KamradtSemanticChunker [14]#
Greg Kamradt proposed a novel semantic chunking algorithm, which was later incorporated into LangChain. The chunker works by first splitting the corpus by sentence. It functions by computing the embedding of a sliding window of tokens over a document, and searching for discontinuities in the cosine distances between consecutive windows. By default, the threshold for detecting a discontinuity is set to any distance above the 95th percentile of all consecutive distances. This is a relative metric and can lead to larger chunks in bigger corpora.
★ KamradtModifiedChunker#
It is desirable for users to be able to set the chunk length directly, in order to ensure that chunks fit within the context window of the embedding model. To address this, we modify the KamradtSemanticChunker
by implementing a binary search over discontinuity thresholds such that the largest chunk is shorter than the specified length.
★ ClusterSemanticChunker#
We further extend the principle of embeddings-based semantic chunking by observing that the KamradtSemanticChunker
algorithm is greedy in nature, which may not produce the optimal chunking under the objective of preserving chunks with high internal similarity. We propose a new algorithm, the ClusterSemanticChunker
, which aims to produce globally optimal chunks by maximizing the sum of cosine similarities within chunks, up to a user-specified maximum length. Intuitively, this preserves as much similar semantic information as possible across the document, while compacting relevant information in each chunk.
Documents are divided into pieces of at most 50 tokens using the RecursiveCharacterTextSplitter
, and pieces are individually embedded. Subsequently, we use a dynamic programming approach to maximize the pairwise cosine similarity between all pairs of pieces within any chunk. Code for this algorithm is available in the GitHub repository
A limitation to this method is that the globally optimal packing relies on the global statistics of the corpus, requiring chunks to be re-computed as data is added. This limitation may be improved upon by maintaining an appropriate data-structure over the smaller pieces as data is added or removed; we leave this to future work.
★ LLMSemanticChunker#
In the spirit of "just [ask] the model", we experimented with directly prompting an LLM to chunk the text. We found that a naive approach where the LLM is prompted to repeat the corpus with a <|split|>
token was costly, slow, and suffered from hallucinations in the produced text.
To resolve this we broke the text into chunks of size 50 in tokens using a RecursiveCharacterTextSplitter
. Then we re-joined the text but surrounded by chunk tags resulting in text like the following:
<start_chunk_0>On glancing over my notes of the seventy odd cases in which I have during the last eight years <end_chunk_0><start_chunk_1>studied the methods of my friend Sherlock Holmes, I find many tragic, some comic, a large number <end_chunk_1><start_chunk_2> . . .
We then instruct the LLM to return the indexes of chunks to split on, in the form
split_after: 3, 5
We found both GPT-4o and Llama 3 8B Instruct consistently adhered to this format. Complete prompts and code for this algorithm are available in the GitHub repository
Results#
We present the mean of Recall, Precision, Precision$_\Omega$, and IoU scores for each chunking method, over all queries and corpora in our evaluation. ± indicates the standard deviation. We report the results for n=5 retrieved chunks, using OpenAI's text-embedding-3-large
as the embedding model.
Chunking | Size | Overlap | Recall | Precision | Precision$_\Omega$ | IoU |
---|---|---|---|---|---|---|
Recursive | 800 (~661) | 400 | 85.4 ± 34.9 | 1.5 ± 1.3 | 6.7 ± 5.2 | 1.5 ± 1.3 |
TokenText | 800 | 400 | 87.9 ± 31.7 | 1.4 ± 1.1 | 4.7 ± 3.1 | 1.4 ± 1.1 |
Recursive | 400 (~312) | 200 | 88.1 ± 31.6 | 3.3 ± 2.7 | 13.9 ± 10.4 | 3.3 ± 2.7 |
TokenText | 400 | 200 | 88.6 ± 29.7 | 2.7 ± 2.2 | 8.4 ± 5.1 | 2.7 ± 2.2 |
Recursive | 400 (~276) | 0 | 89.5 ± 29.7 | 3.6 ± 3.2 | 17.7 ± 14.0 | 3.6 ± 3.2 |
TokenText | 400 | 0 | 89.2 ± 29.2 | 2.7 ± 2.2 | 12.5 ± 8.1 | 2.7 ± 2.2 |
Recursive | 200 (~137) | 0 | 88.1 ± 30.1 | 7.0 ± 5.6 | 29.9 ± 18.4 | 6.9 ± 5.6 |
TokenText | 200 | 0 | 87.0 ± 30.8 | 5.2 ± 4.1 | 21.0 ± 11.9 | 5.1 ± 4.1 |
Kamradt | N/A (~660) | 0 | 83.6 ± 36.8 | 1.5 ± 1.6 | 7.4 ± 10.2 | 1.5 ± 1.6 |
★ KamradtMod | 300 (~397) | 0 | 87.1 ± 31.9 | 2.1 ± 2.0 | 10.5 ± 12.3 | 2.1 ± 2.0 |
★ Cluster | 400 (~182) | 0 | 91.3 ± 25.4 | 4.5 ± 3.4 | 20.7 ± 14.5 | 4.5 ± 3.4 |
★ Cluster | 200 (~103) | 0 | 87.3 ± 29.8 | 8.0 ± 6.0 | 34.0 ± 19.7 | 8.0 ± 6.0 |
★ LLM (GPT4o) | N/A (~240) | 0 | 91.9 ± 26.5 | 3.9 ± 3.2 | 19.9 ± 16.3 | 3.9 ± 3.2 |
Results for text-embedding-3-large. Size denotes the chunk size in tokens (cl100k tokenizer). Size in brackets indicates mean chunk size where it may vary by chunking strategy. Overlap denotes the chunk overlap in tokens. Bold values highlight the best performance in each category.
We find that the heuristic RecursiveCharacterTextSplitter
with chunk size 200 and no overlap performs well. While it does not achieve the best result, it is consistently high performing across all evaluation metrtics. We note that it outperforms TokenTextSplitter
across all metrics for chunk size of 400 or less with no chunk overlap. Interestingly, this does not hold for larger chunk sizes and overlap. Unsurprisingly, reducing chunk overlap improves IoU scores, as this metric penalizes redundant information.
OpenAI Assistants can use file search to improve their response, following the standard Retrieval-Augmented Generation (RAG) process. According to their documentation, the default chunking strategy uses a chunk size of 800 tokens with an overlap of 400 tokens [25]. Assuming the TokenTextSplitter
method is employed, we observe that this setting results in slightly below-average recall and the lowest scores across all other metrics, suggesting particularly poor recall-efficiency tradeoffs.
The ClusterSemanticChunker
, with a max chunk size set to 400 tokens, achieves the second highest recall of 0.913. Dropping the max chunk size to 200 results in average recall but the highest precision, Precision$_\Omega$ , and IoU. The LLMSemanticChunkers
achieves the highest recall of 0.919 while having average scores on the remaining metrics, suggesting LLMs are relatively capable at this task.
The KamradtSemanticChunker
with the default settings scores slightly below average across all metrics, with a recall of 0.836. Recall rises to 0.871 with a similar boost in the remaining metrics when the modifications of the KamradtModifiedChunker
are applied. We note that recall improved despite the mean chunk length dropping.
We speculate that recall could reach a maximum before relevant information is diluted within chunks, making it more difficult to retrieve, while chunks which are too small fail to capture necessary context within a single unit.
We repeat our experiments with the embedding model changed to the Sentence Transformers 'all-MiniLM-L6-v2'[26].
Chunking | Size | Overlap | Recall | Precision | Precision$_Ω$ | IoU |
---|---|---|---|---|---|---|
Recursive | 250 (~180) | 125 | 78.7 ± 39.2 | 4.9 ± 4.5 | 21.9 ± 14.9 | 4.9 ± 4.4 |
TokenText | 250 | 125 | 82.4 ± 36.2 | 3.6 ± 3.1 | 11.4 ± 6.6 | 3.5 ± 3.1 |
Recursive | 250 (~162) | 0 | 78.5 ± 39.5 | 5.4 ± 4.9 | 26.7 ± 18.3 | 5.4 ± 4.9 |
TokenText | 250 | 0 | 77.1 ± 39.3 | 3.3 ± 3.0 | 16.4 ± 10.3 | 3.3 ± 3.0 |
Recursive | 200 (~128) | 0 | 75.7 ± 40.7 | 6.5 ± 6.2 | 31.2 ± 18.4 | 6.5 ± 6.1 |
TokenText | 200 | 0 | 76.6 ± 38.8 | 4.1 ± 3.7 | 19.1 ± 11.0 | 4.1 ± 3.6 |
✒ Cluster | 250 (~168) | 0 | 77.3 ± 38.6 | 6.1 ± 5.1 | 28.6 ± 16.7 | 6.0 ± 5.1 |
✒ Cluster | 200 (~102) | 0 | 75.2 ± 39.9 | 7.2 ± 6.1 | 33.6 ± 20.0 | 7.2 ± 6.0 |
Results for all-MiniLM-L6-v2. Size denotes the chunk size in tokens (cl100k tokenizer). Size in brackets indicates mean chunk size where it may vary by chunking strategy. Overlap denotes the chunk overlap in tokens. Bold values highlight the best performance in each category.
We find that the ClusterSemanticChunker
achieves the highest precision, Precision$_\Omega$ and IoU however has slightly below average recall likely due to its small average chunk size. The TokenTextSplitter
achieves the highest recall of 0.824 when configured with chunk size 250 and chunk overlap of 125. This then drops to 0.771 when chunk overlap is set to zero, suggesting that for smaller context, overlapping chunks are necessary for high recall.
Limitations & Future Work#
One limitation in our synthetic evaluation pipeline is that LLMs tend to generate a specific style of question. We attempt to mitigate this by providing it with its previous questions to encourage new ones, but this sometimes exacerbates its lack of creativity. The metric will be more accurate with more realistic and diverse questions. Future work may experiment with various temperature settings and other prompting techniques.
A limitation of the concrete evaluation we present is its relatively small size, and limited diversity. Future work can easily expand on the evaluation we provide by generating larger datasets from larger corpora. Future work may also explore generating excerpt datasets from benchmarks containing existing queries, which are considered to be diverse and widely representative of many retrieval domains. Future work may also blend human-annotated data with synthetic data to improve the evaluation quality.
Finally, our work does not take into account the time required to run these chunking methods, which can vary from almost instantaneous to tens of minutes in the case of the LLMChunker
, which is an important consideration in practical retrieval settings.
Conclusion#
In this work, we introduced a comprehensive framework for generating domain and dataset-specific evaluations that accurately capture critical properties, such as information density via IoU (Intersection over Union), for retreival as used in practical AI applications. Using this framework, we created a concrete evaluation across several popular domains, enabling robust comparisons of various chunking methods.
Our evaluation of popular chunking strategies demonstrate that this evaluation effectively captures variations in retrieval performance due to the selected chunking strategye. We developed the ClusterSemanticChunker
, an embedding-model aware chunking strategy which produced consistently strong results across the evaluation. We also present a second novel chunking strategy, the LLMSemanticChunker
, which also achieves good performance on this evaluation.
References#
[1] Jacob Devlin Maarten Bosma Gaurav Mishra Adam Roberts Paul Barham Hyung Won Chung Charles Sutton Sebastian Gehrmann et al. Aakanksha Chowdhery, Sharan Narang. Palm: Scaling language modeling with pathways. Journal of Machine Learning Research, 24(240):1–113, 2023.
[2] Patrick Lewis, Ethan Perez, Aleksandra Piktus, Fabio Petroni, Vladimir Karpukhin, Naman Goyal, Heinrich Küttler, Mike Lewis, Wen tau Yih, Tim Rocktäschel, Sebastian Riedel, and Douwe Kiela. Retrieval-augmented generation for knowledge-intensive nlp tasks, 2021
[3] Sergey Brin and Lawrence Page. The anatomy of a large-scale hypertextual web search engine. In Proceedings of the seventh international conference on World Wide Web 7, pages 107–117. Elsevier, 1998.
[4] C.J. van Rijsbergen. A probability ranking principle in information retrieval. Journal of Documentation, 33(4):334– 354, 1977.
[5] S. E. Robertson, S. Walker, M. Beaulieu, M. Gatford, and A. Payne. Okapi at trec-3. In Proceedings of the Third Text REtrieval Conference (TREC-3), pages 109–126. NIST, 1995.
[6] Niklas Muennighoff, Nouamane Tazi, Loïc Magne, and Nils Reimers. Mteb: Massive text embedding benchmark, 2022.
[7] Nandan Thakur, Nils Reimers, Andreas Rücklé, Abhishek Srivastava, and Iryna Gurevych. Beir: A heterogenous benchmark for zero-shot evaluation of information retrieval models, 2021.
[8] Weiyun Wang, Shuibo Zhang, Yiming Ren, Yuchen Duan, Tiantong Li, Shuo Liu, Mengkang Hu, Zhe Chen, Kaipeng Zhang, Lewei Lu, Xizhou Zhu, Ping Luo, Yu Qiao, Jifeng Dai, Wenqi Shao, and Wenhai Wang. Needle in a multimodal haystack, 2024.
[9] Marcin Kaszkiel and Justin Zobel. Passage retrieval revisited. In ACM SIGIR Forum, volume 31, pages 178–185. ACM New York, NY, USA, 1997
[10] Bajaj, P., Campos, D., Craswell, N., Deng, L., Gao, J., Liu, X., Majumder, R., McNamara, A., Mitra, B., Nguyen, T., Rosenberg, M., Song, X., Stoica, A., Tiwary, S., & Wang, T. (2018). MS MARCO: A Human Generated MAchine Reading COmprehension Dataset. arXiv preprint arXiv:1611.09268. https://arxiv.org/abs/1611.09268
[11] Jure Leskovec, Anand Rajaraman, and Jeffrey David Ullman. Mining of massive data sets. Cambridge university press, 2020.
[12] Matouš Eibich, Shivay Nagpal, and Alexander Fred-Ojala. Aragog: Advanced rag output grading, 2024.
[13] Shahul Es, Jithin James, Luis Espinosa-Anke, and Steven Schockaert. Ragas: Automated evaluation of retrieval augmented generation, 2023.
[14] Greg Kamradt. 5 levels of text splitting. https://github.com/FullStackRetrieval-com/ RetrievalTutorials/blob/main/tutorials/LevelsOfTextSplitting/5_Levels_Of_Text_ Splitting.ipynb, 2024. Implementation of Semantic Chunking.
[15] Aurelio AI Labs. Semantic chunkers. https://github.com/aurelio-labs/semantic-chunkers, 2024. Library for semantic text chunking.
[16] Antonio Jimeno Yepes, Yao You, Jan Milczek, Sebastian Laverde, and Renyu Li. Financial report chunking for effective retrieval augmented generation, 2024.
[17] Freda Shi, Xinyun Chen, Kanishka Misra, Nathan Scales, David Dohan, Ed Chi, Nathanael Schärli, and Denny Zhou. Large language models can be easily distracted by irrelevant context, 2023.
[18] Md Atiqur Rahman and Yang Wang. Optimizing intersection-over-union in deep neural networks for image segmentation. In George Bebis, Richard Boyle, Bahram Parvin, Darko Koracin, Fatih Porikli, Sandra Skaff, Alireza Entezari, Jianyuan Min, Daisuke Iwai, Amela Sadagic, Carlos Scheidegger, and Tobias Isenberg, editors, Advances in Visual Computing. ISVC 2016. Lecture Notes in Computer Science, volume 10072, pages 234–244. Springer, Cham, 2016.
[19] The White House. State of the union 2024, 2024. Accessed: 2024-05-02.
[20] Stephen Merity, Caiming Xiong, James Bradbury, and Richard Socher. Pointer sentinel mixture models, 2016.
[21] Ning Ding, Yulin Chen, Bokai Xu, Yujia Qin, Zhi Zheng, Shengding Hu, Zhiyuan Liu, Maosong Sun, and Bowen Zhou. Enhancing chat language models by scaling high-quality instructional conversations, 2023.
[22] Zhiyu Chen, Shiyang Li, Charese Smiley, Zhiqiang Ma, Sameena Shah, and William Yang Wang. Convfinqa: Exploring the chain of numerical reasoning in conversational finance question answering. In EMNLP, pages 6279–6292. Association for Computational Linguistics, 2022.
[23] National Library of Medicine. Pmc open access subset. Dataset retrieved from Hugging Face Datasets (2023). PubMed Central Open Access dataset (Version 2023-06-17.commercial). Available from https://huggingface.co/datasets/pmc/open_access, 2003–. [cited 2024 May 08].
[24] LangChain. Recursive text splitter, 2023. Accessed: 2024-04-16.
[25] OpenAI. Vector stores. https://platform.openai.com/docs/assistants/tools/file-search/ vector-stores, 2024. Accessed: 2024-06-27.
[26] HuggingFace. Sentence Transformers, all-MiniLM-L6-v2. https://huggingface.co/sentence-transformers/all-MiniLM-L6-v2 2024. Accessed: 2024-06-27.
[27] Kristian Georgiev Aleksander Madry Benjamin Cohen-Wang, Harshay Shah. Contextcite: Attributing model generation to context. https://github.com/MadryLab/context-cite/tree/main, 2024.
Appendices#
Synthetic Dataset Prompt#
You are an agent that generates questions from provided text. Your job is to generate a question and provide the relevant sections from the text as references.
Instructions:
- For each provided text, generate a question that can be answered solely by the facts in the text.
- Extract all significant facts that answer the generated question.
- Format the response in JSON format with two fields:
- 'question': A question directly related to these facts, ensuring it can only be answered using the references provided.
- 'references': A list of all text sections that answer the generated question. These must be exact copies from the original text and should be whole sentences where possible.
Notes: Make the question more specific. Do not ask a question about multiple topics. Do not ask a question with over 5 references.
Example:
Text: "Experiment A: The temperature control test showed that at higher temperatures, the reaction rate increased significantly, resulting in quicker product formation. However, at extremely high temperatures, the reaction yield decreased due to the degradation of reactants.
Experiment B: The pH sensitivity test revealed that the reaction is highly dependent on acidity, with optimal results at a pH of 7. Deviating from this pH level in either direction led to a substantial drop in yield.
Experiment C: In the enzyme activity assay, it was found that the presence of a specific enzyme accelerated the reaction by a factor of 3. The absence of the enzyme, however, led to a sluggish reaction with an extended completion time.
Experiment D: The light exposure trial demonstrated that UV light stimulated the reaction, making it complete in half the time compared to the absence of light. Conversely, prolonged light exposure led to unwanted side reactions that contaminated the final product."
Response: { 'oath': "I will not use the word 'and' in the question unless it is part of a proper noun. I will also make sure the question is concise.", 'question': 'What experiments were done in this paper?', 'references': ['Experiment A: The temperature control test showed that at higher temperatures, the reaction rate increased significantly, resulting in quicker product formation.', 'Experiment B: The pH sensitivity test revealed that the reaction is highly dependent on acidity, with optimal results at a pH of 7.', 'Experiment C: In the enzyme activity assay, it was found that the presence of a specific enzyme accelerated the reaction by a factor of 3.', 'Experiment D: The light exposure trial demonstrated that UV light stimulated the reaction, making it complete in half the time compared to the absence of light.'] }
DO NOT USE THE WORD 'and' IN THE QUESTION UNLESS IT IS PART OF A PROPER NOUN. YOU MUST INCLUDE THE OATH ABOVE IN YOUR RESPONSE. YOU MUST ALSO NOT REPEAT A QUESTION THAT HAS ALREADY BEEN USED."
An interesting observation from the above is the inclusion of an oath. We require these queries to be specific so that the excerpts answer only that query. This generally means the query shouldn't include 'and' unless it is part of a proper noun. Despite this requirement being part of the prompt at many points, GPT would not comply until we required it to include an 'oath': "I will not use the word 'and' in the question unless it is part of a proper noun. I will also make sure the question is concise." in the JSON response. It then consistently included the oath and followed our requirements.
Further Algorithms#
Logit & Attention Based Chunking#
We experimented with next token prediction probabilities and attention values to determine if any signals could be used for chunking. All these experiments were conducted using Llama 3 8B, which provides access to next token logits and attention values.
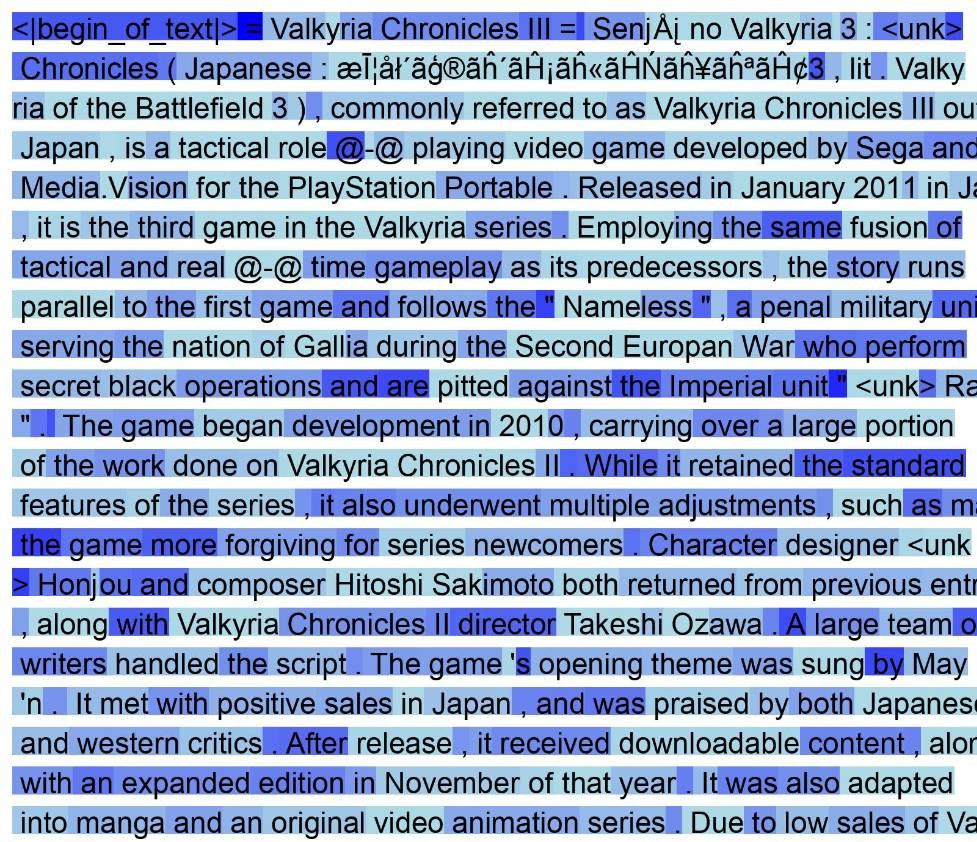
Token entropy visualization over Wikitext corpus using Llama 3 8B. The color gradient from light blue (under 1 bit) to dark blue (8 bits and over) indicates the entropy in the next token prediction at that token.
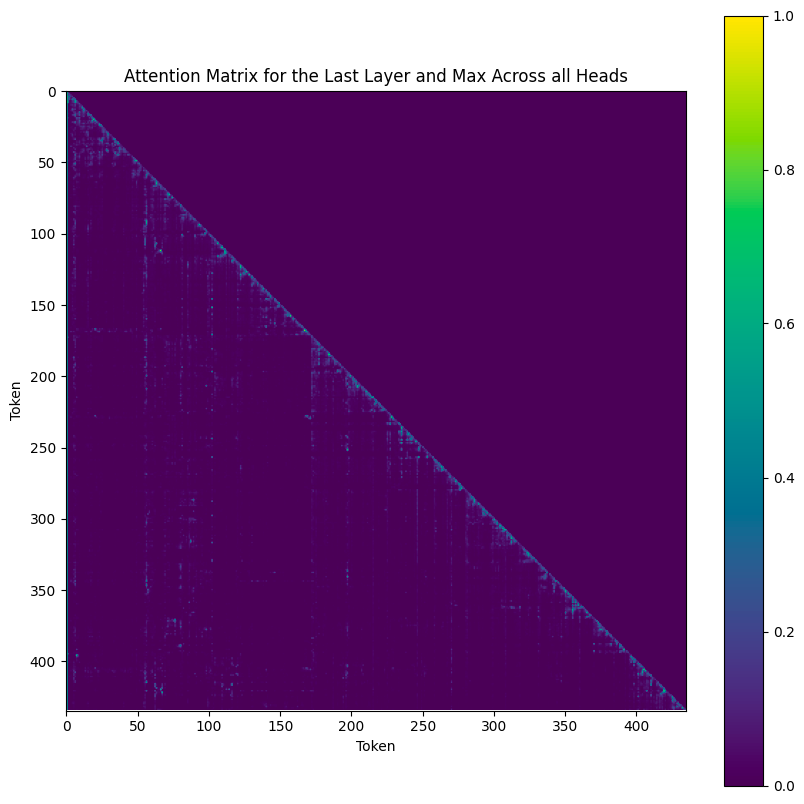
Heatmap of attention values from the Llama 3 8B model on the wikitext corpus. This plot shows the max attention values in the last layer across all 32 heads, with x and y axes representing token positions.
We calculate token entropy according to:
$$H(Y) = -\sum_{t} P(y_t) \log P(y_t)$$
Where $Y$ is a random variable representing the next token, $y_t$ is the $t$-th possible token and $P(y_t)$ is the probability assigned to token $(y_t)$. We observe that in general, token entropy rises at the beginning and towards the end of sentences, as well as after a verb after which any noun or adjective could be expected. We initially expected that the entropy would rise as the theme of the text changes, however, we find that the LLM quickly refocuses between topics and so we did not extract a clear signal for semantic boundaries.
We also did not find that there was any clear structure in the attention values which could be used for chunking.
ContextCite Inspired Chunking#
The final algorithm was inspired by ContextCite [27]. Their work leverages next-token prediction to observe how the probability of an LLM generating a response changes as different parts of the input prompt are masked. This allows them to fit weights to each part of the input prompt, determining how much it influences the likelihood of generating a specific response. Essentially, this results in a weighted importance of each part of the input prompt relative to the response.
For our work, we split the Wikitext corpus into 50-token chunks using a RecursiveCharacterTextSplitter
and applied the same algorithm, but with each chunk as the 'response' and all previous chunks as the input prompt. This allowed us to fit weights between each chunk. Unfortunately, no clear structure emerged, and we were unable to derive a useful chunking algorithm.
All Results#
We present the mean of Recall, Precision, Precision$_\Omega$, and IoU scores for each chunking method, over all queries and corpora in our evaluation. ± indicates the standard deviation. We vary the number of chunks retrieved: 'Min' retrieves the minimum number of chunks containing excerpts (usually 1-3), while 5 and 10 represent fixed retrieval numbers for all queries. Captions indicate corpus and embedding model.
Chunking | Size | Overlap | Retrieve | Recall | Precision | Precision_Ω | IoU |
---|---|---|---|---|---|---|---|
Recursive | 800 | 400 | Min | 66.7 ± 46.6 | 3.5 ± 4.4 | 6.7 ± 5.2 | 3.5 ± 4.4 |
TokenText | 800 | 400 | Min | 75.6 ± 41.8 | 2.6 ± 2.4 | 4.7 ± 3.1 | 2.6 ± 2.4 |
Recursive | 400 | 200 | Min | 73.3 ± 43.4 | 8.5 ± 10.0 | 13.9 ± 10.4 | 8.4 ± 10.0 |
TokenText | 400 | 200 | Min | 77.9 ± 39.8 | 4.6 ± 3.8 | 8.4 ± 5.1 | 4.5 ± 3.8 |
Recursive | 400 | 0 | Min | 61.4 ± 47.7 | 10.8 ± 13.2 | 17.7 ± 14.0 | 10.8 ± 13.2 |
TokenText | 400 | 0 | Min | 58.1 ± 47.5 | 7.2 ± 8.5 | 12.5 ± 8.1 | 7.1 ± 8.5 |
Recursive | 200 | 0 | Min | 70.2 ± 42.8 | 20.4 ± 20.0 | 29.9 ± 18.4 | 20.2 ± 20.0 |
TokenText | 200 | 0 | Min | 66.7 ± 43.3 | 13.4 ± 12.9 | 21.0 ± 11.9 | 13.3 ± 12.9 |
Cluster | 400 | 0 | Min | 68.6 ± 44.8 | 11.8 ± 12.7 | 17.8 ± 13.2 | 11.7 ± 12.7 |
Cluster | 200 | 0 | Min | 69.3 ± 43.0 | 19.1 ± 18.2 | 29.1 ± 17.0 | 18.9 ± 18.2 |
LLM | N/A | 0 | Min | 65.4 ± 46.7 | 13.1 ± 16.2 | 19.9 ± 16.3 | 13.0 ± 16.2 |
Recursive | 800 | 400 | 5 | 85.4 ± 34.9 | 1.5 ± 1.3 | 6.7 ± 5.2 | 1.5 ± 1.3 |
TokenText | 800 | 400 | 5 | 88.5 ± 30.9 | 1.4 ± 1.1 | 4.7 ± 3.1 | 1.4 ± 1.1 |
Recursive | 400 | 200 | 5 | 88.3 ± 31.3 | 3.3 ± 2.7 | 13.9 ± 10.4 | 3.3 ± 2.7 |
TokenText | 400 | 200 | 5 | 87.3 ± 31.3 | 2.6 ± 2.2 | 8.4 ± 5.1 | 2.6 ± 2.2 |
Recursive | 400 | 0 | 5 | 89.9 ± 29.1 | 3.6 ± 3.2 | 17.7 ± 14.0 | 3.6 ± 3.2 |
TokenText | 400 | 0 | 5 | 89.7 ± 28.6 | 2.7 ± 2.2 | 12.5 ± 8.1 | 2.7 ± 2.2 |
Recursive | 200 | 0 | 5 | 88.5 ± 29.5 | 7.0 ± 5.6 | 29.9 ± 18.4 | 7.0 ± 5.6 |
TokenText | 200 | 0 | 5 | 86.7 ± 31.0 | 5.1 ± 4.1 | 21.0 ± 11.9 | 5.1 ± 4.1 |
Cluster | 400 | 0 | 5 | 92.1 ± 25.2 | 3.7 ± 2.7 | 17.8 ± 13.2 | 3.7 ± 2.7 |
Cluster | 200 | 0 | 5 | 89.0 ± 28.9 | 6.7 ± 4.9 | 29.1 ± 17.0 | 6.6 ± 4.9 |
LLM | N/A | 0 | 5 | 91.7 ± 26.8 | 3.9 ± 3.2 | 19.9 ± 16.3 | 3.9 ± 3.2 |
Recursive | 800 | 400 | 10 | 91.5 ± 27.5 | 0.8 ± 0.7 | 6.7 ± 5.2 | 0.8 ± 0.7 |
TokenText | 800 | 400 | 10 | 94.8 ± 21.7 | 0.7 ± 0.6 | 4.7 ± 3.1 | 0.7 ± 0.6 |
Recursive | 400 | 200 | 10 | 94.5 ± 22.0 | 1.7 ± 1.3 | 13.9 ± 10.4 | 1.7 ± 1.3 |
TokenText | 400 | 200 | 10 | 92.5 ± 25.0 | 1.4 ± 1.1 | 8.4 ± 5.1 | 1.4 ± 1.1 |
Recursive | 400 | 0 | 10 | 94.5 ± 21.8 | 1.9 ± 1.5 | 17.7 ± 14.0 | 1.9 ± 1.5 |
TokenText | 400 | 0 | 10 | 95.1 ± 20.6 | 1.5 ± 1.1 | 12.5 ± 8.1 | 1.5 ± 1.1 |
Recursive | 200 | 0 | 10 | 92.8 ± 24.3 | 3.7 ± 2.7 | 29.9 ± 18.4 | 3.7 ± 2.7 |
TokenText | 200 | 0 | 10 | 93.1 ± 23.0 | 2.8 ± 2.1 | 21.0 ± 11.9 | 2.8 ± 2.1 |
Cluster | 400 | 0 | 10 | 96.2 ± 17.6 | 2.0 ± 1.4 | 17.8 ± 13.2 | 2.0 ± 1.4 |
Cluster | 200 | 0 | 10 | 93.7 ± 22.4 | 3.6 ± 2.5 | 29.1 ± 17.0 | 3.6 ± 2.5 |
LLM | N/A | 0 | 10 | 95.7 ± 19.8 | 2.0 ± 1.5 | 19.9 ± 16.3 | 2.0 ± 1.5 |
All corpora, text-embedding-3-large
Chunking | Size | Overlap | Retrieve | Recall | Precision | Precision_Ω | IoU |
---|---|---|---|---|---|---|---|
Recursive | 800 | 400 | Min | 80.8 ± 39.0 | 4.8 ± 4.4 | 7.2 ± 4.7 | 4.8 ± 4.4 |
TokenText | 800 | 400 | Min | 89.5 ± 28.6 | 3.5 ± 2.5 | 5.4 ± 3.5 | 3.5 ± 2.5 |
Recursive | 400 | 200 | Min | 94.3 ± 22.6 | 8.0 ± 4.6 | 11.4 ± 6.4 | 8.0 ± 4.6 |
TokenText | 400 | 200 | Min | 82.2 ± 37.6 | 5.8 ± 4.5 | 10.0 ± 5.9 | 5.8 ± 4.5 |
Recursive | 400 | 0 | Min | 76.5 ± 41.4 | 13.2 ± 11.2 | 16.1 ± 9.5 | 13.2 ± 11.2 |
TokenText | 400 | 0 | Min | 67.1 ± 44.5 | 10.2 ± 10.0 | 14.1 ± 8.2 | 10.2 ± 10.0 |
Recursive | 200 | 0 | Min | 86.7 ± 30.1 | 23.5 ± 14.0 | 25.7 ± 12.2 | 23.4 ± 14.1 |
TokenText | 200 | 0 | Min | 77.3 ± 37.7 | 19.5 ± 14.8 | 24.7 ± 13.2 | 19.2 ± 14.7 |
Cluster | 400 | 0 | Min | 78.5 ± 39.7 | 14.2 ± 11.3 | 16.7 ± 9.6 | 14.2 ± 11.3 |
Cluster | 200 | 0 | Min | 85.6 ± 31.4 | 23.3 ± 14.8 | 25.8 ± 12.4 | 23.2 ± 14.9 |
LLM | N/A | 0 | Min | 74.0 ± 42.1 | 16.1 ± 16.2 | 19.3 ± 15.1 | 16.0 ± 16.2 |
Recursive | 800 | 400 | 5 | 94.6 ± 22.5 | 1.9 ± 1.6 | 7.2 ± 4.7 | 1.9 ± 1.6 |
TokenText | 800 | 400 | 5 | 94.4 ± 21.3 | 1.7 ± 1.4 | 5.4 ± 3.5 | 1.7 ± 1.4 |
Recursive | 400 | 200 | 5 | 99.6 ± 2.7 | 3.9 ± 2.9 | 11.4 ± 6.4 | 3.9 ± 2.9 |
TokenText | 400 | 200 | 5 | 87.1 ± 32.2 | 3.1 ± 2.9 | 10.0 ± 5.9 | 3.1 ± 2.9 |
Recursive | 400 | 0 | 5 | 100.0 ± 0.0 | 4.1 ± 3.1 | 16.1 ± 9.5 | 4.1 ± 3.1 |
TokenText | 400 | 0 | 5 | 94.4 ± 21.3 | 3.5 ± 2.9 | 14.1 ± 8.2 | 3.5 ± 2.9 |
Recursive | 200 | 0 | 5 | 99.6 ± 2.7 | 8.3 ± 6.6 | 25.7 ± 12.2 | 8.3 ± 6.5 |
TokenText | 200 | 0 | 5 | 89.0 ± 30.9 | 6.4 ± 5.7 | 24.7 ± 13.2 | 6.4 ± 5.7 |
Cluster | 400 | 0 | 5 | 100.0 ± 0.1 | 4.1 ± 3.1 | 16.7 ± 9.6 | 4.1 ± 3.1 |
Cluster | 200 | 0 | 5 | 99.1 ± 3.8 | 7.4 ± 5.5 | 25.8 ± 12.4 | 7.3 ± 5.5 |
LLM | N/A | 0 | 5 | 95.9 ± 18.8 | 5.0 ± 3.9 | 19.3 ± 15.1 | 5.0 ± 3.9 |
Recursive | 800 | 400 | 10 | 94.6 ± 22.5 | 1.0 ± 0.8 | 7.2 ± 4.7 | 1.0 ± 0.8 |
TokenText | 800 | 400 | 10 | 98.7 ± 9.5 | 1.0 ± 0.7 | 5.4 ± 3.5 | 1.0 ± 0.7 |
Recursive | 400 | 200 | 10 | 100.0 ± 0.0 | 2.0 ± 1.5 | 11.4 ± 6.4 | 2.0 ± 1.5 |
TokenText | 400 | 200 | 10 | 89.3 ± 30.9 | 1.6 ± 1.5 | 10.0 ± 5.9 | 1.6 ± 1.5 |
Recursive | 400 | 0 | 10 | 100.0 ± 0.0 | 2.2 ± 1.6 | 16.1 ± 9.5 | 2.2 ± 1.6 |
TokenText | 400 | 0 | 10 | 98.2 ± 13.2 | 1.9 ± 1.5 | 14.1 ± 8.2 | 1.9 ± 1.5 |
Recursive | 200 | 0 | 10 | 100.0 ± 0.0 | 4.3 ± 3.2 | 25.7 ± 12.2 | 4.3 ± 3.2 |
TokenText | 200 | 0 | 10 | 89.3 ± 30.9 | 3.2 ± 2.9 | 24.7 ± 13.2 | 3.2 ± 2.9 |
Cluster | 400 | 0 | 10 | 100.0 ± 0.1 | 2.5 ± 1.9 | 16.7 ± 9.6 | 2.5 ± 1.9 |
Cluster | 200 | 0 | 10 | 99.8 ± 0.8 | 3.9 ± 2.9 | 25.8 ± 12.4 | 3.9 ± 2.9 |
LLM | N/A | 0 | 10 | 100.0 ± 0.1 | 2.5 ± 1.8 | 19.3 ± 15.1 | 2.5 ± 1.8 |
Chatlogs corpus, text-embedding-3-large
Chunking | Size | Overlap | Retrieve | Recall | Precision | Precision_Ω | IoU |
---|---|---|---|---|---|---|---|
Recursive | 800 | 400 | Min | 53.8 ± 49.1 | 2.9 ± 4.3 | 6.9 ± 6.0 | 2.9 ± 4.3 |
TokenText | 800 | 400 | Min | 62.8 ± 47.2 | 1.8 ± 2.1 | 3.9 ± 3.0 | 1.8 ± 2.1 |
Recursive | 400 | 200 | Min | 64.4 ± 46.9 | 5.3 ± 7.4 | 12.0 ± 9.2 | 5.3 ± 7.4 |
TokenText | 400 | 200 | Min | 78.1 ± 40.5 | 3.3 ± 3.5 | 7.0 ± 5.3 | 3.3 ± 3.5 |
Recursive | 400 | 0 | Min | 54.3 ± 48.3 | 7.5 ± 12.3 | 17.4 ± 18.7 | 7.5 ± 12.3 |
TokenText | 400 | 0 | Min | 55.9 ± 48.8 | 4.8 ± 6.6 | 10.0 ± 8.0 | 4.8 ± 6.6 |
Recursive | 200 | 0 | Min | 61.5 ± 46.6 | 12.8 ± 16.4 | 27.1 ± 18.6 | 12.8 ± 16.4 |
TokenText | 200 | 0 | Min | 64.9 ± 45.7 | 8.6 ± 11.4 | 17.2 ± 13.2 | 8.6 ± 11.4 |
Cluster | 400 | 0 | Min | 58.9 ± 48.3 | 6.6 ± 9.3 | 14.0 ± 10.8 | 6.6 ± 9.3 |
Cluster | 200 | 0 | Min | 63.5 ± 47.1 | 11.6 ± 14.5 | 24.4 ± 17.1 | 11.6 ± 14.4 |
LLM | N/A | 0 | Min | 49.4 ± 49.5 | 5.9 ± 9.7 | 13.6 ± 11.6 | 5.9 ± 9.7 |
Recursive | 800 | 400 | 5 | 74.2 ± 43.2 | 1.1 ± 1.3 | 6.9 ± 6.0 | 1.1 ± 1.3 |
TokenText | 800 | 400 | 5 | 79.3 ± 40.4 | 1.0 ± 1.1 | 3.9 ± 3.0 | 1.0 ± 1.1 |
Recursive | 400 | 200 | 5 | 81.3 ± 37.5 | 2.7 ± 2.5 | 12.0 ± 9.2 | 2.7 ± 2.5 |
TokenText | 400 | 200 | 5 | 86.0 ± 34.0 | 2.2 ± 2.1 | 7.0 ± 5.3 | 2.2 ± 2.1 |
Recursive | 400 | 0 | 5 | 84.0 ± 35.4 | 3.4 ± 4.3 | 17.4 ± 18.7 | 3.4 ± 4.3 |
TokenText | 400 | 0 | 5 | 83.6 ± 35.5 | 2.1 ± 2.1 | 10.0 ± 8.0 | 2.1 ± 2.1 |
Recursive | 200 | 0 | 5 | 79.6 ± 38.4 | 5.9 ± 5.6 | 27.1 ± 18.6 | 5.9 ± 5.6 |
TokenText | 200 | 0 | 5 | 83.7 ± 35.3 | 4.2 ± 4.0 | 17.2 ± 13.2 | 4.2 ± 3.9 |
Cluster | 400 | 0 | 5 | 85.1 ± 33.9 | 2.8 ± 2.3 | 14.0 ± 10.8 | 2.8 ± 2.3 |
Cluster | 200 | 0 | 5 | 82.1 ± 37.7 | 5.3 ± 4.8 | 24.4 ± 17.1 | 5.3 ± 4.8 |
LLM | N/A | 0 | 5 | 85.2 ± 35.2 | 3.3 ± 3.0 | 13.6 ± 11.6 | 3.3 ± 3.0 |
Recursive | 800 | 400 | 10 | 83.0 ± 37.2 | 0.6 ± 0.6 | 6.9 ± 6.0 | 0.6 ± 0.6 |
TokenText | 800 | 400 | 10 | 88.4 ± 31.2 | 0.6 ± 0.6 | 3.9 ± 3.0 | 0.6 ± 0.6 |
Recursive | 400 | 200 | 10 | 91.5 ± 27.5 | 1.4 ± 1.1 | 12.0 ± 9.2 | 1.4 ± 1.1 |
TokenText | 400 | 200 | 10 | 94.2 ± 22.4 | 1.2 ± 1.0 | 7.0 ± 5.3 | 1.2 ± 1.0 |
Recursive | 400 | 0 | 10 | 91.0 ± 27.8 | 1.7 ± 1.6 | 17.4 ± 18.7 | 1.7 ± 1.6 |
TokenText | 400 | 0 | 10 | 86.2 ± 33.0 | 1.1 ± 1.1 | 10.0 ± 8.0 | 1.1 ± 1.1 |
Recursive | 200 | 0 | 10 | 88.0 ± 31.0 | 3.0 ± 2.5 | 27.1 ± 18.6 | 3.0 ± 2.5 |
TokenText | 200 | 0 | 10 | 91.1 ± 27.7 | 2.3 ± 2.0 | 17.2 ± 13.2 | 2.3 ± 2.0 |
Cluster | 400 | 0 | 10 | 95.8 ± 18.6 | 1.6 ± 1.1 | 14.0 ± 10.8 | 1.6 ± 1.1 |
Cluster | 200 | 0 | 10 | 87.8 ± 31.7 | 2.9 ± 2.4 | 24.4 ± 17.1 | 2.9 ± 2.4 |
LLM | N/A | 0 | 10 | 92.4 ± 26.0 | 1.7 ± 1.4 | 13.6 ± 11.6 | 1.7 ± 1.4 |
Finance corpus, text-embedding-3-large
Chunking | Size | Overlap | Retrieve | Recall | Precision | Precision_Ω | IoU |
---|---|---|---|---|---|---|---|
Recursive | 800 | 400 | Min | 51.2 ± 49.1 | 4.0 ± 6.5 | 9.1 ± 6.6 | 4.0 ± 6.5 |
TokenText | 800 | 400 | Min | 66.0 ± 45.0 | 3.2 ± 3.1 | 6.1 ± 3.6 | 3.2 ± 3.1 |
Recursive | 400 | 200 | Min | 58.3 ± 47.9 | 11.5 ± 14.4 | 19.6 ± 13.5 | 11.5 ± 14.4 |
TokenText | 400 | 200 | Min | 68.6 ± 43.0 | 5.1 ± 4.4 | 10.6 ± 5.4 | 5.1 ± 4.4 |
Recursive | 400 | 0 | Min | 51.6 ± 48.8 | 13.0 ± 17.2 | 23.3 ± 16.5 | 13.0 ± 17.2 |
TokenText | 400 | 0 | Min | 49.6 ± 46.6 | 8.1 ± 10.7 | 16.0 ± 9.7 | 8.0 ± 10.7 |
Recursive | 200 | 0 | Min | 54.9 ± 43.7 | 21.7 ± 23.2 | 36.4 ± 19.5 | 21.1 ± 23.3 |
TokenText | 200 | 0 | Min | 47.2 ± 43.6 | 10.8 ± 12.1 | 24.3 ± 11.4 | 10.5 ± 11.9 |
Cluster | 400 | 0 | Min | 50.7 ± 46.9 | 12.4 ± 17.0 | 24.9 ± 19.6 | 12.3 ± 17.0 |
Cluster | 200 | 0 | Min | 61.9 ± 42.8 | 22.1 ± 22.1 | 36.7 ± 20.0 | 21.6 ± 22.1 |
LLM | N/A | 0 | Min | 57.0 ± 48.0 | 15.1 ± 20.7 | 27.2 ± 20.6 | 15.1 ± 20.7 |
Recursive | 800 | 400 | 5 | 80.3 ± 38.7 | 1.9 ± 1.6 | 9.1 ± 6.6 | 1.9 ± 1.6 |
TokenText | 800 | 400 | 5 | 81.5 ± 36.2 | 1.8 ± 1.4 | 6.1 ± 3.6 | 1.8 ± 1.4 |
Recursive | 400 | 200 | 5 | 80.3 ± 38.6 | 4.2 ± 3.6 | 19.6 ± 13.5 | 4.2 ± 3.6 |
TokenText | 400 | 200 | 5 | 82.5 ± 33.5 | 3.3 ± 2.5 | 10.6 ± 5.4 | 3.3 ± 2.5 |
Recursive | 400 | 0 | 5 | 83.6 ± 35.3 | 4.6 ± 3.6 | 23.3 ± 16.5 | 4.6 ± 3.5 |
TokenText | 400 | 0 | 5 | 77.2 ± 38.4 | 3.2 ± 2.6 | 16.0 ± 9.7 | 3.2 ± 2.6 |
Recursive | 200 | 0 | 5 | 81.1 ± 33.2 | 9.0 ± 6.8 | 36.4 ± 19.5 | 8.9 ± 6.8 |
TokenText | 200 | 0 | 5 | 75.7 ± 37.1 | 5.9 ± 4.6 | 24.3 ± 11.4 | 5.9 ± 4.6 |
Cluster | 400 | 0 | 5 | 82.5 ± 35.3 | 4.5 ± 3.3 | 24.9 ± 19.6 | 4.4 ± 3.3 |
Cluster | 200 | 0 | 5 | 81.7 ± 33.4 | 8.1 ± 5.7 | 36.7 ± 20.0 | 8.0 ± 5.6 |
LLM | N/A | 0 | 5 | 86.3 ± 32.1 | 4.6 ± 3.6 | 27.2 ± 20.6 | 4.6 ± 3.6 |
Recursive | 800 | 400 | 10 | 85.9 ± 34.2 | 1.1 ± 0.8 | 9.1 ± 6.6 | 1.1 ± 0.8 |
TokenText | 800 | 400 | 10 | 91.4 ± 27.4 | 1.0 ± 0.7 | 6.1 ± 3.6 | 1.0 ± 0.7 |
Recursive | 400 | 200 | 10 | 89.8 ± 28.3 | 2.3 ± 1.7 | 19.6 ± 13.5 | 2.3 ± 1.7 |
TokenText | 400 | 200 | 10 | 89.6 ± 26.6 | 1.8 ± 1.3 | 10.6 ± 5.4 | 1.8 ± 1.3 |
Recursive | 400 | 0 | 10 | 89.2 ± 28.7 | 2.4 ± 1.7 | 23.3 ± 16.5 | 2.4 ± 1.7 |
TokenText | 400 | 0 | 10 | 92.3 ± 24.9 | 1.9 ± 1.3 | 16.0 ± 9.7 | 1.9 ± 1.3 |
Recursive | 200 | 0 | 10 | 88.2 ± 28.2 | 4.9 ± 3.3 | 36.4 ± 19.5 | 4.8 ± 3.3 |
TokenText | 200 | 0 | 10 | 90.6 ± 23.2 | 3.6 ± 2.3 | 24.3 ± 11.4 | 3.5 ± 2.3 |
Cluster | 400 | 0 | 10 | 89.4 ± 29.0 | 2.4 ± 1.7 | 24.9 ± 19.6 | 2.4 ± 1.7 |
Cluster | 200 | 0 | 10 | 91.1 ± 23.7 | 4.5 ± 2.8 | 36.7 ± 20.0 | 4.5 ± 2.8 |
LLM | N/A | 0 | 10 | 95.4 ± 19.9 | 2.5 ± 1.6 | 27.2 ± 20.6 | 2.5 ± 1.6 |
Pubmed corpus, text-embedding-3-large
Chunking | Size | Overlap | Retrieve | Recall | Precision | Precision_Ω | IoU |
---|---|---|---|---|---|---|---|
Recursive | 800 | 400 | Min | 82.9 ± 37.6 | 2.2 ± 1.7 | 3.6 ± 2.1 | 2.2 ± 1.7 |
TokenText | 800 | 400 | Min | 88.6 ± 31.3 | 2.3 ± 1.7 | 3.3 ± 2.1 | 2.3 ± 1.7 |
Recursive | 400 | 200 | Min | 93.4 ± 24.8 | 5.1 ± 3.2 | 7.1 ± 3.9 | 5.1 ± 3.2 |
TokenText | 400 | 200 | Min | 87.2 ± 32.4 | 4.1 ± 2.9 | 6.2 ± 3.6 | 4.1 ± 2.9 |
Recursive | 400 | 0 | Min | 67.1 ± 46.3 | 7.3 ± 8.0 | 10.6 ± 6.9 | 7.3 ± 8.0 |
TokenText | 400 | 0 | Min | 71.1 ± 45.3 | 7.0 ± 7.2 | 9.4 ± 6.2 | 7.0 ± 7.2 |
Recursive | 200 | 0 | Min | 88.9 ± 30.8 | 19.5 ± 12.9 | 21.3 ± 11.7 | 19.5 ± 12.9 |
TokenText | 200 | 0 | Min | 83.7 ± 35.5 | 15.0 ± 11.1 | 16.8 ± 9.6 | 15.0 ± 11.1 |
Cluster | 400 | 0 | Min | 88.6 ± 30.7 | 14.3 ± 12.1 | 15.9 ± 11.4 | 14.3 ± 12.1 |
Cluster | 200 | 0 | Min | 84.3 ± 35.0 | 24.1 ± 18.7 | 28.5 ± 16.9 | 24.0 ± 18.6 |
LLM | N/A | 0 | Min | 84.3 ± 35.8 | 15.0 ± 15.2 | 18.0 ± 15.4 | 15.0 ± 15.2 |
Recursive | 800 | 400 | 5 | 92.1 ± 27.0 | 1.0 ± 0.7 | 3.6 ± 2.1 | 1.0 ± 0.7 |
TokenText | 800 | 400 | 5 | 96.1 ± 19.5 | 1.0 ± 0.7 | 3.3 ± 2.1 | 1.0 ± 0.7 |
Recursive | 400 | 200 | 5 | 97.4 ± 16.0 | 2.2 ± 1.4 | 7.1 ± 3.9 | 2.2 ± 1.4 |
TokenText | 400 | 200 | 5 | 94.7 ± 22.3 | 2.0 ± 1.3 | 6.2 ± 3.6 | 2.0 ± 1.3 |
Recursive | 400 | 0 | 5 | 94.7 ± 22.3 | 2.1 ± 1.5 | 10.6 ± 6.9 | 2.1 ± 1.5 |
TokenText | 400 | 0 | 5 | 98.7 ± 11.4 | 2.0 ± 1.3 | 9.4 ± 6.2 | 2.0 ± 1.3 |
Recursive | 200 | 0 | 5 | 98.6 ± 11.4 | 4.5 ± 2.9 | 21.3 ± 11.7 | 4.5 ± 2.9 |
TokenText | 200 | 0 | 5 | 95.7 ± 19.6 | 3.9 ± 2.6 | 16.8 ± 9.6 | 3.9 ± 2.6 |
Cluster | 400 | 0 | 5 | 98.3 ± 11.5 | 3.4 ± 2.3 | 15.9 ± 11.4 | 3.4 ± 2.3 |
Cluster | 200 | 0 | 5 | 97.6 ± 12.1 | 6.1 ± 4.1 | 28.5 ± 16.9 | 6.1 ± 4.1 |
LLM | N/A | 0 | 5 | 97.3 ± 16.0 | 3.3 ± 3.0 | 18.0 ± 15.4 | 3.3 ± 3.0 |
Recursive | 800 | 400 | 10 | 94.7 ± 22.3 | 0.5 ± 0.4 | 3.6 ± 2.1 | 0.5 ± 0.4 |
TokenText | 800 | 400 | 10 | 98.7 ± 11.4 | 0.5 ± 0.3 | 3.3 ± 2.1 | 0.5 ± 0.3 |
Recursive | 400 | 200 | 10 | 98.7 ± 11.4 | 1.1 ± 0.7 | 7.1 ± 3.9 | 1.1 ± 0.7 |
TokenText | 400 | 200 | 10 | 97.4 ± 16.0 | 1.0 ± 0.7 | 6.2 ± 3.6 | 1.0 ± 0.7 |
Recursive | 400 | 0 | 10 | 97.3 ± 16.0 | 1.1 ± 0.7 | 10.6 ± 6.9 | 1.1 ± 0.7 |
TokenText | 400 | 0 | 10 | 100.0 ± 0.0 | 1.0 ± 0.6 | 9.4 ± 6.2 | 1.0 ± 0.6 |
Recursive | 200 | 0 | 10 | 98.6 ± 11.4 | 2.3 ± 1.4 | 21.3 ± 11.7 | 2.3 ± 1.4 |
TokenText | 200 | 0 | 10 | 97.4 ± 16.0 | 2.0 ± 1.3 | 16.8 ± 9.6 | 2.0 ± 1.3 |
Cluster | 400 | 0 | 10 | 98.3 ± 11.5 | 1.7 ± 1.1 | 15.9 ± 11.4 | 1.7 ± 1.1 |
Cluster | 200 | 0 | 10 | 98.1 ± 11.5 | 3.0 ± 2.0 | 28.5 ± 16.9 | 3.0 ± 2.0 |
LLM | N/A | 0 | 10 | 97.3 ± 16.0 | 1.6 ± 1.1 | 18.0 ± 15.4 | 1.6 ± 1.1 |
State of the Union corpus, text-embedding-3-large
Chunking | Size | Overlap | Retrieve | Recall | Precision | Precision_Ω | IoU |
---|---|---|---|---|---|---|---|
Recursive | 800 | 400 | Min | 72.1 ± 44.5 | 3.6 ± 3.5 | 6.3 ± 3.9 | 3.6 ± 3.5 |
TokenText | 800 | 400 | Min | 78.6 ± 40.5 | 2.7 ± 2.0 | 4.6 ± 2.4 | 2.7 ± 2.0 |
Recursive | 400 | 200 | Min | 71.0 ± 44.6 | 10.4 ± 10.7 | 16.0 ± 9.7 | 10.4 ± 10.7 |
TokenText | 400 | 200 | Min | 77.6 ± 40.1 | 4.8 ± 3.4 | 8.5 ± 4.1 | 4.8 ± 3.4 |
Recursive | 400 | 0 | Min | 64.1 ± 47.2 | 12.5 ± 12.6 | 18.6 ± 10.6 | 12.5 ± 12.6 |
TokenText | 400 | 0 | Min | 55.1 ± 47.7 | 7.1 ± 7.5 | 12.8 ± 6.7 | 7.0 ± 7.5 |
Recursive | 200 | 0 | Min | 70.3 ± 43.3 | 24.0 ± 23.2 | 33.5 ± 19.9 | 23.8 ± 23.2 |
TokenText | 200 | 0 | Min | 68.1 ± 41.8 | 15.3 ± 13.0 | 21.9 ± 10.3 | 15.1 ± 13.0 |
Cluster | 400 | 0 | Min | 72.8 ± 42.7 | 12.5 ± 11.1 | 16.8 ± 8.9 | 12.4 ± 11.1 |
Cluster | 200 | 0 | Min | 64.0 ± 44.2 | 17.8 ± 16.5 | 28.6 ± 14.1 | 17.6 ± 16.5 |
LLM | N/A | 0 | Min | 68.5 ± 45.9 | 14.3 ± 15.2 | 20.3 ± 14.5 | 14.2 ± 15.1 |
Recursive | 800 | 400 | 5 | 89.3 ± 30.7 | 1.6 ± 1.1 | 6.3 ± 3.9 | 1.6 ± 1.1 |
TokenText | 800 | 400 | 5 | 93.3 ± 24.5 | 1.4 ± 0.9 | 4.6 ± 2.4 | 1.4 ± 0.9 |
Recursive | 400 | 200 | 5 | 89.4 ± 30.6 | 3.4 ± 2.3 | 16.0 ± 9.7 | 3.4 ± 2.3 |
TokenText | 400 | 200 | 5 | 87.6 ± 30.8 | 2.6 ± 1.7 | 8.5 ± 4.1 | 2.6 ± 1.7 |
Recursive | 400 | 0 | 5 | 91.9 ± 26.7 | 3.6 ± 2.4 | 18.6 ± 10.6 | 3.6 ± 2.4 |
TokenText | 400 | 0 | 5 | 95.7 ± 18.9 | 2.8 ± 1.7 | 12.8 ± 6.7 | 2.8 ± 1.7 |
Recursive | 200 | 0 | 5 | 90.0 ± 29.0 | 7.1 ± 4.6 | 33.5 ± 19.9 | 7.1 ± 4.6 |
TokenText | 200 | 0 | 5 | 90.8 ± 25.3 | 5.4 ± 3.3 | 21.9 ± 10.3 | 5.4 ± 3.3 |
Cluster | 400 | 0 | 5 | 97.1 ± 14.8 | 3.8 ± 2.1 | 16.8 ± 8.9 | 3.8 ± 2.1 |
Cluster | 200 | 0 | 5 | 90.1 ± 27.9 | 6.6 ± 4.2 | 28.6 ± 14.1 | 6.6 ± 4.2 |
LLM | N/A | 0 | 5 | 95.1 ± 21.5 | 3.9 ± 2.4 | 20.3 ± 14.5 | 3.9 ± 2.4 |
Recursive | 800 | 400 | 10 | 98.3 ± 12.3 | 0.9 ± 0.5 | 6.3 ± 3.9 | 0.9 ± 0.5 |
TokenText | 800 | 400 | 10 | 97.8 ± 14.4 | 0.7 ± 0.4 | 4.6 ± 2.4 | 0.7 ± 0.4 |
Recursive | 400 | 200 | 10 | 95.5 ± 20.3 | 1.8 ± 1.1 | 16.0 ± 9.7 | 1.8 ± 1.1 |
TokenText | 400 | 200 | 10 | 91.9 ± 26.2 | 1.3 ± 0.9 | 8.5 ± 4.1 | 1.3 ± 0.9 |
Recursive | 400 | 0 | 10 | 96.8 ± 16.8 | 1.9 ± 1.2 | 18.6 ± 10.6 | 1.9 ± 1.2 |
TokenText | 400 | 0 | 10 | 99.2 ± 8.5 | 1.5 ± 0.8 | 12.8 ± 6.7 | 1.5 ± 0.8 |
Recursive | 200 | 0 | 10 | 93.3 ± 24.4 | 3.8 ± 2.3 | 33.5 ± 19.9 | 3.8 ± 2.3 |
TokenText | 200 | 0 | 10 | 95.5 ± 17.8 | 2.8 ± 1.6 | 21.9 ± 10.3 | 2.8 ± 1.6 |
Cluster | 400 | 0 | 10 | 98.7 ± 9.3 | 2.0 ± 1.1 | 16.8 ± 8.9 | 2.0 ± 1.1 |
Cluster | 200 | 0 | 10 | 94.7 ± 21.5 | 3.6 ± 2.2 | 28.6 ± 14.1 | 3.6 ± 2.2 |
LLM | N/A | 0 | 10 | 95.8 ± 20.0 | 2.0 ± 1.3 | 20.3 ± 14.5 | 2.0 ± 1.3 |
Wikitext corpus, text-embedding-3-large
Chunking | Size | Overlap | Retrieve | Recall | Precision | Precision_Ω | IoU |
---|---|---|---|---|---|---|---|
Recursive | 250 | 125 | Min | 60.7 ± 47.4 | 10.6 ± 14.1 | 21.9 ± 14.9 | 10.6 ± 14.0 |
TokenText | 250 | 125 | Min | 73.4 ± 42.6 | 5.6 ± 4.9 | 11.4 ± 6.6 | 5.6 ± 4.9 |
Recursive | 250 | 0 | Min | 54.1 ± 47.9 | 14.6 ± 19.1 | 26.7 ± 18.3 | 14.4 ± 19.1 |
TokenText | 250 | 0 | Min | 52.4 ± 46.7 | 8.5 ± 10.6 | 16.4 ± 10.3 | 8.4 ± 10.6 |
Recursive | 200 | 0 | Min | 48.5 ± 47.3 | 14.5 ± 19.1 | 31.2 ± 18.4 | 14.3 ± 19.0 |
TokenText | 200 | 0 | Min | 53.6 ± 46.0 | 10.0 ± 11.9 | 19.1 ± 11.0 | 9.9 ± 11.9 |
Cluster | 250 | 0 | Min | 55.7 ± 46.5 | 14.4 ± 16.9 | 28.6 ± 16.7 | 14.1 ± 16.8 |
Cluster | 200 | 0 | Min | 51.9 ± 46.8 | 15.8 ± 19.1 | 33.6 ± 20.0 | 15.5 ± 19.0 |
Recursive | 250 | 125 | 5 | 78.7 ± 39.2 | 4.9 ± 4.5 | 21.9 ± 14.9 | 4.9 ± 4.4 |
TokenText | 250 | 125 | 5 | 82.4 ± 36.2 | 3.6 ± 3.1 | 11.4 ± 6.6 | 3.5 ± 3.1 |
Recursive | 250 | 0 | 5 | 78.5 ± 39.5 | 5.4 ± 4.9 | 26.7 ± 18.3 | 5.4 ± 4.9 |
TokenText | 250 | 0 | 5 | 77.1 ± 39.3 | 3.3 ± 3.0 | 16.4 ± 10.3 | 3.3 ± 3.0 |
Recursive | 200 | 0 | 5 | 75.7 ± 40.7 | 6.5 ± 6.2 | 31.2 ± 18.4 | 6.5 ± 6.1 |
TokenText | 200 | 0 | 5 | 76.6 ± 38.8 | 4.1 ± 3.7 | 19.1 ± 11.0 | 4.1 ± 3.6 |
Cluster | 250 | 0 | 5 | 77.3 ± 38.6 | 6.1 ± 5.1 | 28.6 ± 16.7 | 6.0 ± 5.1 |
Cluster | 200 | 0 | 5 | 75.2 ± 39.9 | 7.2 ± 6.1 | 33.6 ± 20.0 | 7.2 ± 6.0 |
Recursive | 250 | 125 | 10 | 87.2 ± 32.3 | 2.8 ± 2.3 | 21.9 ± 14.9 | 2.8 ± 2.3 |
TokenText | 250 | 125 | 10 | 88.4 ± 30.8 | 1.9 ± 1.6 | 11.4 ± 6.6 | 1.9 ± 1.6 |
Recursive | 250 | 0 | 10 | 84.7 ± 34.5 | 2.9 ± 2.5 | 26.7 ± 18.3 | 2.9 ± 2.5 |
TokenText | 250 | 0 | 10 | 85.7 ± 33.1 | 1.9 ± 1.6 | 16.4 ± 10.3 | 1.9 ± 1.6 |
Recursive | 200 | 0 | 10 | 83.7 ± 35.0 | 3.7 ± 3.0 | 31.2 ± 18.4 | 3.7 ± 3.0 |
TokenText | 200 | 0 | 10 | 85.4 ± 32.3 | 2.4 ± 1.9 | 19.1 ± 11.0 | 2.4 ± 1.9 |
Cluster | 250 | 0 | 10 | 84.4 ± 33.3 | 3.5 ± 2.7 | 28.6 ± 16.7 | 3.5 ± 2.7 |
Cluster | 200 | 0 | 10 | 84.4 ± 32.8 | 4.2 ± 3.2 | 33.6 ± 20.0 | 4.2 ± 3.2 |
All corpora, all-MiniLM-L6-v2
Chunking | Size | Overlap | Retrieve | Recall | Precision | Precision_Ω | IoU |
---|---|---|---|---|---|---|---|
Recursive | 250 | 125 | Min | 91.1 ± 24.6 | 12.5 ± 6.8 | 19.0 ± 9.2 | 12.5 ± 6.8 |
TokenText | 250 | 125 | Min | 92.9 ± 25.8 | 8.8 ± 5.5 | 13.3 ± 7.9 | 8.8 ± 5.5 |
Recursive | 250 | 0 | Min | 70.5 ± 42.5 | 18.7 ± 16.0 | 25.0 ± 12.7 | 18.5 ± 16.0 |
TokenText | 250 | 0 | Min | 76.3 ± 37.0 | 15.9 ± 13.0 | 18.8 ± 11.1 | 15.8 ± 13.0 |
Recursive | 200 | 0 | Min | 67.0 ± 41.1 | 22.1 ± 18.9 | 32.9 ± 16.3 | 21.5 ± 18.8 |
TokenText | 200 | 0 | Min | 77.0 ± 37.5 | 19.6 ± 14.1 | 23.0 ± 11.1 | 19.5 ± 14.2 |
Cluster | 250 | 0 | Min | 77.0 ± 37.7 | 20.7 ± 16.4 | 27.6 ± 14.0 | 20.5 ± 16.5 |
Cluster | 200 | 0 | Min | 68.6 ± 42.2 | 22.4 ± 18.8 | 34.1 ± 19.6 | 21.9 ± 18.8 |
Recursive | 250 | 125 | 5 | 94.6 ± 17.4 | 7.1 ± 4.7 | 19.0 ± 9.2 | 7.0 ± 4.4 |
TokenText | 250 | 125 | 5 | 100.0 ± 0.0 | 5.3 ± 4.0 | 13.3 ± 7.9 | 5.3 ± 4.0 |
Recursive | 250 | 0 | 5 | 98.2 ± 13.2 | 7.6 ± 5.8 | 25.0 ± 12.7 | 7.6 ± 5.8 |
TokenText | 250 | 0 | 5 | 97.7 ± 13.5 | 5.1 ± 3.9 | 18.8 ± 11.1 | 5.1 ± 3.9 |
Recursive | 200 | 0 | 5 | 96.5 ± 15.7 | 9.2 ± 7.3 | 32.9 ± 16.3 | 9.2 ± 7.3 |
TokenText | 200 | 0 | 5 | 98.7 ± 7.1 | 6.5 ± 4.9 | 23.0 ± 11.1 | 6.4 ± 4.9 |
Cluster | 250 | 0 | 5 | 95.9 ± 15.0 | 8.4 ± 6.4 | 27.6 ± 14.0 | 8.3 ± 6.4 |
Cluster | 200 | 0 | 5 | 91.1 ± 23.0 | 9.7 ± 6.7 | 34.1 ± 19.6 | 9.5 ± 6.2 |
Recursive | 250 | 125 | 10 | 98.2 ± 13.2 | 3.8 ± 3.0 | 19.0 ± 9.2 | 3.8 ± 3.0 |
TokenText | 250 | 125 | 10 | 100.0 ± 0.0 | 2.6 ± 2.0 | 13.3 ± 7.9 | 2.6 ± 2.0 |
Recursive | 250 | 0 | 10 | 98.2 ± 13.2 | 4.0 ± 3.0 | 25.0 ± 12.7 | 4.0 ± 3.0 |
TokenText | 250 | 0 | 10 | 97.9 ± 13.4 | 2.6 ± 2.1 | 18.8 ± 11.1 | 2.6 ± 2.1 |
Recursive | 200 | 0 | 10 | 100.0 ± 0.0 | 4.9 ± 3.6 | 32.9 ± 16.3 | 4.9 ± 3.6 |
TokenText | 200 | 0 | 10 | 100.0 ± 0.0 | 3.3 ± 2.6 | 23.0 ± 11.1 | 3.3 ± 2.6 |
Cluster | 250 | 0 | 10 | 99.1 ± 4.5 | 4.5 ± 3.2 | 27.6 ± 14.0 | 4.5 ± 3.2 |
Cluster | 200 | 0 | 10 | 97.6 ± 9.1 | 5.3 ± 4.0 | 34.1 ± 19.6 | 5.3 ± 4.0 |
Chatlogs corpus, all-MiniLM-L6-v2
Chunking | Size | Overlap | Retrieve | Recall | Precision | Precision_Ω | IoU |
---|---|---|---|---|---|---|---|
Recursive | 250 | 125 | Min | 59.0 ± 48.7 | 7.1 ± 10.1 | 18.8 ± 12.3 | 7.1 ± 10.1 |
TokenText | 250 | 125 | Min | 72.4 ± 43.1 | 3.7 ± 3.9 | 9.4 ± 6.7 | 3.7 ± 3.9 |
Recursive | 250 | 0 | Min | 50.0 ± 48.5 | 9.5 ± 15.8 | 23.1 ± 17.9 | 9.4 ± 15.8 |
TokenText | 250 | 0 | Min | 55.5 ± 47.6 | 5.3 ± 7.1 | 12.8 ± 9.3 | 5.3 ± 7.1 |
Recursive | 200 | 0 | Min | 49.4 ± 47.7 | 9.3 ± 12.6 | 26.3 ± 16.4 | 9.2 ± 12.5 |
TokenText | 200 | 0 | Min | 57.4 ± 46.6 | 5.9 ± 7.4 | 14.5 ± 10.4 | 5.9 ± 7.3 |
Cluster | 250 | 0 | Min | 55.3 ± 48.2 | 9.0 ± 12.4 | 25.2 ± 17.0 | 8.9 ± 12.4 |
Cluster | 200 | 0 | Min | 51.2 ± 47.8 | 9.9 ± 13.3 | 29.5 ± 21.9 | 9.8 ± 13.2 |
Recursive | 250 | 125 | 5 | 69.3 ± 44.9 | 3.7 ± 3.7 | 18.8 ± 12.3 | 3.7 ± 3.7 |
TokenText | 250 | 125 | 5 | 79.1 ± 39.3 | 3.0 ± 2.9 | 9.4 ± 6.7 | 3.0 ± 2.9 |
Recursive | 250 | 0 | 5 | 69.7 ± 44.2 | 4.2 ± 4.0 | 23.1 ± 17.9 | 4.2 ± 4.0 |
TokenText | 250 | 0 | 5 | 72.8 ± 42.1 | 2.7 ± 2.7 | 12.8 ± 9.3 | 2.7 ± 2.7 |
Recursive | 200 | 0 | 5 | 71.3 ± 43.1 | 5.8 ± 6.0 | 26.3 ± 16.4 | 5.7 ± 5.8 |
TokenText | 200 | 0 | 5 | 73.4 ± 41.5 | 3.5 ± 3.5 | 14.5 ± 10.4 | 3.5 ± 3.4 |
Cluster | 250 | 0 | 5 | 74.9 ± 41.6 | 4.9 ± 4.5 | 25.2 ± 17.0 | 4.9 ± 4.5 |
Cluster | 200 | 0 | 5 | 69.6 ± 43.7 | 5.9 ± 5.7 | 29.5 ± 21.9 | 5.8 ± 5.7 |
Recursive | 250 | 125 | 10 | 79.2 ± 40.4 | 2.2 ± 2.0 | 18.8 ± 12.3 | 2.2 ± 2.0 |
TokenText | 250 | 125 | 10 | 87.0 ± 32.9 | 1.6 ± 1.5 | 9.4 ± 6.7 | 1.6 ± 1.5 |
Recursive | 250 | 0 | 10 | 77.6 ± 40.3 | 2.4 ± 2.2 | 23.1 ± 17.9 | 2.4 ± 2.2 |
TokenText | 250 | 0 | 10 | 77.0 ± 40.3 | 1.5 ± 1.4 | 12.8 ± 9.3 | 1.4 ± 1.4 |
Recursive | 200 | 0 | 10 | 79.3 ± 38.7 | 3.1 ± 2.8 | 26.3 ± 16.4 | 3.1 ± 2.8 |
TokenText | 200 | 0 | 10 | 78.2 ± 38.5 | 1.9 ± 1.8 | 14.5 ± 10.4 | 1.9 ± 1.8 |
Cluster | 250 | 0 | 10 | 78.7 ± 38.9 | 2.6 ± 2.4 | 25.2 ± 17.0 | 2.6 ± 2.4 |
Cluster | 200 | 0 | 10 | 79.8 ± 38.8 | 3.3 ± 3.0 | 29.5 ± 21.9 | 3.3 ± 3.0 |
Finance corpus, all-MiniLM-L6-v2
Chunking | Size | Overlap | Retrieve | Recall | Precision | Precision_Ω | IoU |
---|---|---|---|---|---|---|---|
Recursive | 250 | 125 | Min | 42.8 ± 46.6 | 11.2 ± 18.1 | 28.8 ± 18.5 | 11.1 ± 18.0 |
TokenText | 250 | 125 | Min | 61.4 ± 46.3 | 5.9 ± 5.5 | 14.1 ± 7.1 | 5.9 ± 5.5 |
Recursive | 250 | 0 | Min | 37.2 ± 45.1 | 13.4 ± 20.9 | 34.0 ± 21.1 | 13.1 ± 20.8 |
TokenText | 250 | 0 | Min | 45.4 ± 45.7 | 9.8 ± 12.6 | 20.8 ± 12.2 | 9.6 ± 12.6 |
Recursive | 200 | 0 | Min | 31.7 ± 43.1 | 12.7 ± 21.0 | 38.1 ± 21.5 | 12.3 ± 20.7 |
TokenText | 200 | 0 | Min | 37.5 ± 42.8 | 8.6 ± 11.8 | 23.0 ± 11.5 | 8.4 ± 11.7 |
Cluster | 250 | 0 | Min | 42.7 ± 44.2 | 13.1 ± 16.2 | 33.4 ± 17.1 | 12.6 ± 15.9 |
Cluster | 200 | 0 | Min | 35.1 ± 43.7 | 11.8 ± 17.9 | 38.7 ± 19.8 | 11.5 ± 17.8 |
Recursive | 250 | 125 | 5 | 63.7 ± 45.2 | 5.9 ± 6.2 | 28.8 ± 18.5 | 5.8 ± 6.2 |
TokenText | 250 | 125 | 5 | 68.4 ± 44.1 | 3.9 ± 3.5 | 14.1 ± 7.1 | 3.9 ± 3.5 |
Recursive | 250 | 0 | 5 | 62.4 ± 45.3 | 6.6 ± 6.6 | 34.0 ± 21.1 | 6.6 ± 6.6 |
TokenText | 250 | 0 | 5 | 63.1 ± 44.2 | 3.6 ± 3.5 | 20.8 ± 12.2 | 3.6 ± 3.5 |
Recursive | 200 | 0 | 5 | 61.0 ± 45.7 | 7.9 ± 8.0 | 38.1 ± 21.5 | 7.8 ± 7.9 |
TokenText | 200 | 0 | 5 | 61.3 ± 43.3 | 4.4 ± 4.1 | 23.0 ± 11.5 | 4.3 ± 4.1 |
Cluster | 250 | 0 | 5 | 61.3 ± 43.1 | 6.3 ± 5.8 | 33.4 ± 17.1 | 6.2 ± 5.7 |
Cluster | 200 | 0 | 5 | 59.8 ± 43.9 | 7.1 ± 6.4 | 38.7 ± 19.8 | 6.9 ± 6.3 |
Recursive | 250 | 125 | 10 | 74.6 ± 41.1 | 3.5 ± 3.0 | 28.8 ± 18.5 | 3.5 ± 3.0 |
TokenText | 250 | 125 | 10 | 76.8 ± 40.7 | 2.3 ± 1.9 | 14.1 ± 7.1 | 2.3 ± 1.9 |
Recursive | 250 | 0 | 10 | 69.6 ± 43.4 | 3.6 ± 3.2 | 34.0 ± 21.1 | 3.6 ± 3.2 |
TokenText | 250 | 0 | 10 | 73.0 ± 41.0 | 2.2 ± 1.9 | 20.8 ± 12.2 | 2.2 ± 1.9 |
Recursive | 200 | 0 | 10 | 67.2 ± 43.4 | 4.3 ± 3.8 | 38.1 ± 21.5 | 4.3 ± 3.8 |
TokenText | 200 | 0 | 10 | 73.6 ± 39.6 | 2.7 ± 2.2 | 23.0 ± 11.5 | 2.7 ± 2.2 |
Cluster | 250 | 0 | 10 | 71.5 ± 40.6 | 3.9 ± 3.3 | 33.4 ± 17.1 | 3.9 ± 3.3 |
Cluster | 200 | 0 | 10 | 73.5 ± 38.2 | 4.5 ± 3.5 | 38.7 ± 19.8 | 4.4 ± 3.4 |
Pubmed corpus, all-MiniLM-L6-v2
Chunking | Size | Overlap | Retrieve | Recall | Precision | Precision_Ω | IoU |
---|---|---|---|---|---|---|---|
Recursive | 250 | 125 | Min | 71.9 ± 44.6 | 7.7 ± 7.0 | 13.2 ± 7.0 | 7.7 ± 7.0 |
TokenText | 250 | 125 | Min | 78.3 ± 40.8 | 4.8 ± 4.1 | 8.6 ± 4.9 | 4.8 ± 4.1 |
Recursive | 250 | 0 | Min | 62.5 ± 47.5 | 13.2 ± 14.2 | 18.7 ± 11.0 | 13.1 ± 14.2 |
TokenText | 250 | 0 | Min | 52.4 ± 47.8 | 6.8 ± 8.4 | 13.0 ± 8.2 | 6.8 ± 8.4 |
Recursive | 200 | 0 | Min | 51.6 ± 48.7 | 12.7 ± 14.9 | 23.0 ± 11.6 | 12.6 ± 14.8 |
TokenText | 200 | 0 | Min | 53.1 ± 46.6 | 7.8 ± 9.5 | 14.2 ± 8.2 | 7.7 ± 9.5 |
Cluster | 250 | 0 | Min | 63.3 ± 45.2 | 13.9 ± 15.9 | 22.2 ± 14.2 | 13.8 ± 15.9 |
Cluster | 200 | 0 | Min | 55.6 ± 47.4 | 14.8 ± 16.5 | 27.8 ± 15.7 | 14.5 ± 16.4 |
Recursive | 250 | 125 | 5 | 91.2 ± 27.2 | 3.7 ± 2.7 | 13.2 ± 7.0 | 3.7 ± 2.7 |
TokenText | 250 | 125 | 5 | 88.7 ± 31.2 | 2.6 ± 2.0 | 8.6 ± 4.9 | 2.6 ± 2.0 |
Recursive | 250 | 0 | 5 | 82.5 ± 37.6 | 3.6 ± 3.0 | 18.7 ± 11.0 | 3.6 ± 3.0 |
TokenText | 250 | 0 | 5 | 82.0 ± 36.5 | 2.4 ± 2.0 | 13.0 ± 8.2 | 2.4 ± 2.0 |
Recursive | 200 | 0 | 5 | 82.7 ± 36.5 | 4.6 ± 3.7 | 23.0 ± 11.6 | 4.6 ± 3.7 |
TokenText | 200 | 0 | 5 | 80.5 ± 36.5 | 2.9 ± 2.5 | 14.2 ± 8.2 | 2.9 ± 2.5 |
Cluster | 250 | 0 | 5 | 85.0 ± 32.3 | 5.4 ± 4.3 | 22.2 ± 14.2 | 5.4 ± 4.3 |
Cluster | 200 | 0 | 5 | 85.0 ± 33.6 | 6.9 ± 6.3 | 27.8 ± 15.7 | 6.9 ± 6.3 |
Recursive | 250 | 125 | 10 | 96.4 ± 16.9 | 2.0 ± 1.3 | 13.2 ± 7.0 | 2.0 ± 1.3 |
TokenText | 250 | 125 | 10 | 94.7 ± 22.3 | 1.4 ± 1.0 | 8.6 ± 4.9 | 1.4 ± 1.0 |
Recursive | 250 | 0 | 10 | 87.3 ± 32.4 | 1.9 ± 1.4 | 18.7 ± 11.0 | 1.9 ± 1.4 |
TokenText | 250 | 0 | 10 | 90.3 ± 28.3 | 1.3 ± 1.0 | 13.0 ± 8.2 | 1.3 ± 1.0 |
Recursive | 200 | 0 | 10 | 88.7 ± 30.9 | 2.5 ± 1.9 | 23.0 ± 11.6 | 2.5 ± 1.9 |
TokenText | 200 | 0 | 10 | 89.3 ± 27.0 | 1.6 ± 1.2 | 14.2 ± 8.2 | 1.6 ± 1.2 |
Cluster | 250 | 0 | 10 | 90.1 ± 27.2 | 3.0 ± 2.3 | 22.2 ± 14.2 | 3.0 ± 2.3 |
Cluster | 200 | 0 | 10 | 88.5 ± 29.1 | 3.7 ± 3.0 | 27.8 ± 15.7 | 3.7 ± 3.0 |
State of the Union corpus, all-MiniLM-L6-v2
Chunking | Size | Overlap | Retrieve | Recall | Precision | Precision_Ω | IoU |
---|---|---|---|---|---|---|---|
Recursive | 250 | 125 | Min | 56.3 ± 48.3 | 13.4 ± 17.0 | 24.8 ± 15.4 | 13.4 ± 17.0 |
TokenText | 250 | 125 | Min | 72.0 ± 42.8 | 5.8 ± 4.4 | 11.5 ± 5.2 | 5.7 ± 4.4 |
Recursive | 250 | 0 | Min | 57.7 ± 47.8 | 17.9 ± 22.0 | 29.1 ± 19.0 | 17.8 ± 22.0 |
TokenText | 250 | 0 | Min | 45.9 ± 46.3 | 7.7 ± 9.4 | 16.7 ± 8.4 | 7.6 ± 9.4 |
Recursive | 200 | 0 | Min | 50.5 ± 47.8 | 17.3 ± 21.8 | 33.3 ± 18.6 | 17.1 ± 21.8 |
TokenText | 200 | 0 | Min | 53.3 ± 45.9 | 11.0 ± 12.6 | 20.5 ± 10.2 | 10.9 ± 12.6 |
Cluster | 250 | 0 | Min | 52.8 ± 47.2 | 16.7 ± 19.4 | 31.4 ± 16.9 | 16.4 ± 19.1 |
Cluster | 200 | 0 | Min | 55.4 ± 46.2 | 20.6 ± 22.3 | 35.8 ± 19.7 | 20.2 ± 22.3 |
Recursive | 250 | 125 | 5 | 82.7 ± 36.9 | 4.9 ± 3.7 | 24.8 ± 15.4 | 4.9 ± 3.7 |
TokenText | 250 | 125 | 5 | 84.2 ± 33.6 | 3.6 ± 2.5 | 11.5 ± 5.2 | 3.6 ± 2.5 |
Recursive | 250 | 0 | 5 | 85.7 ± 33.5 | 5.4 ± 3.8 | 29.1 ± 19.0 | 5.4 ± 3.8 |
TokenText | 250 | 0 | 5 | 79.1 ± 37.6 | 3.3 ± 2.6 | 16.7 ± 8.4 | 3.3 ± 2.6 |
Recursive | 200 | 0 | 5 | 76.9 ± 40.0 | 6.0 ± 4.6 | 33.3 ± 18.6 | 5.9 ± 4.6 |
TokenText | 200 | 0 | 5 | 78.7 ± 37.1 | 4.1 ± 2.9 | 20.5 ± 10.2 | 4.1 ± 2.9 |
Cluster | 250 | 0 | 5 | 78.7 ± 38.2 | 6.2 ± 4.6 | 31.4 ± 16.9 | 6.1 ± 4.6 |
Cluster | 200 | 0 | 5 | 78.1 ± 38.2 | 7.5 ± 5.4 | 35.8 ± 19.7 | 7.4 ± 5.4 |
Recursive | 250 | 125 | 10 | 92.1 ± 25.8 | 2.8 ± 1.8 | 24.8 ± 15.4 | 2.8 ± 1.8 |
TokenText | 250 | 125 | 10 | 89.5 ± 28.3 | 1.9 ± 1.3 | 11.5 ± 5.2 | 1.9 ± 1.3 |
Recursive | 250 | 0 | 10 | 93.1 ± 23.8 | 3.0 ± 1.9 | 29.1 ± 19.0 | 3.0 ± 1.9 |
TokenText | 250 | 0 | 10 | 93.2 ± 23.9 | 2.0 ± 1.2 | 16.7 ± 8.4 | 2.0 ± 1.2 |
Recursive | 200 | 0 | 10 | 89.0 ± 29.5 | 3.7 ± 2.5 | 33.3 ± 18.6 | 3.7 ± 2.5 |
TokenText | 200 | 0 | 10 | 90.8 ± 26.5 | 2.5 ± 1.6 | 20.5 ± 10.2 | 2.5 ± 1.6 |
Cluster | 250 | 0 | 10 | 88.5 ± 28.7 | 3.7 ± 2.4 | 31.4 ± 16.9 | 3.7 ± 2.3 |
Cluster | 200 | 0 | 10 | 87.6 ± 29.1 | 4.3 ± 2.6 | 35.8 ± 19.7 | 4.3 ± 2.6 |
Wikitext, all-MiniLM-L6-v2
An example query and set of excerpts generated from the Wikitexts corpus, one of the 474 queries in our generated dataset, sampled from GPT-4-Turbo in JSON mode. Excerpts are exact matches from the Wikitexts corpus.